MLCommons releases MLPerf Training 4.0 results and unveils two new benchmarks
MLCommons released MLPerf Training v4.0 showcasing major performance gains. Remarkably, NVIDIA set new records using software optimizations on its Hopper architecture. MLPerf Training 4.0 introduces novel benchmarks for LoRA-based fine-tuning and graph neural network training.
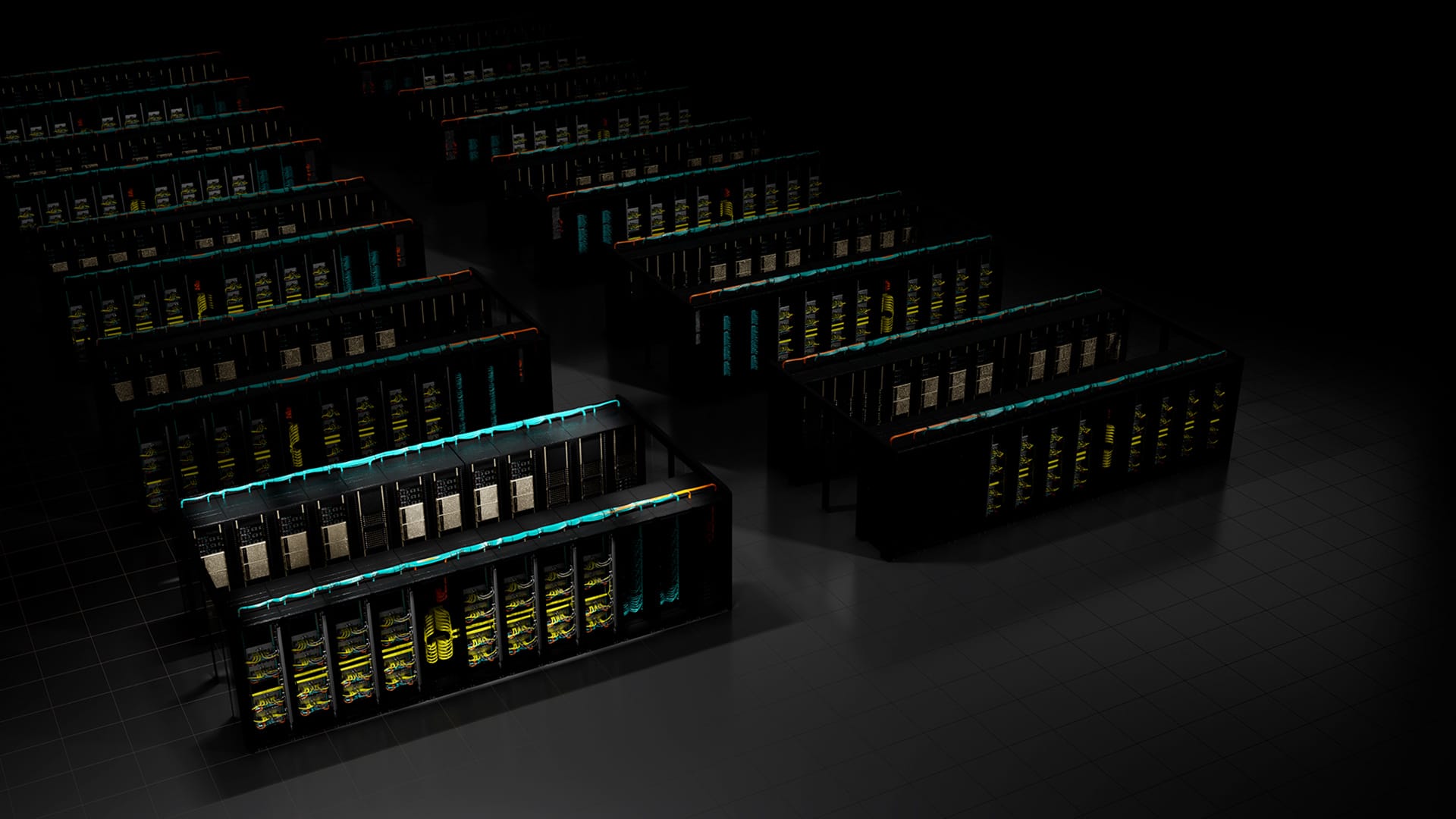
MLCommons has released the latest MLPerf Training v4.0 benchmark suite results, showcasing substantial performance gains across machine learning workloads from various participants. This edition of the MLPerft Training counted with over 200 performance results from 17 submitters, including newcomers Juniper Networks, Oracle, SMC, and tiny corp. Unsurprisingly, nearly all of the most notable results belong to NVIDIA, with a couple of intermissions by Google's TPU-v5p, and a single appearance of Intel's Gaudi 2, and AMD's Radeon RX 7900 XTX.
Something remarkable about NVIDIA's feat is that in addition to claiming to have set new generative AI training performance records, it has done so using the Hopper architecture, the same core hardware platform it tested for the MLPerf Training 3.1 results submitted last year. Perhaps unexpectedly, the MLPerf Training became the ideal stage for NVIDIA to showcase its capabilities to deliver enhanced hardware performance by delivering continued software innovation. The software improvements involved in the latest MLPerf Training results include optimized FP8 kernels, a new FP8-aware distributed optimizer, an optimized FlashAttention implementation in cuDNN, more effective overlapped execution of math operations and GPU-to-GPU communication operations, and intelligent power allocation within the H100 GPUs to maximize Tensor Core throughput.
The latest edition of the MLPerf Training also saw the addition of two new benchmarks, one for low-rank adaptation (LoRA) fine-tuning, and one targeting graph neural networks (GNNs) training. The benchmark uses the 70B parameter LLama 2 model and the Scrolls dataset to evaluate document summarization quality using the ROUGE metric. The GNN benchmark evaluates performance on multi-label node classification with nearly 3,000 classes. MLPerf Training team submitted the R-GAT model underlying this benchmark to the Illinois Graph Benchmark (IGB) leaderboard, which the R-GAT model currently tops for accuracy, with a 72% rating.