Activeloop announces its Series A with a reflection on the future of AI data
Activeloop, the AI data infrastructure company behind Deep Lake, celebrates its $11M Series A with an illuminating reflection on AI data's past, present, and future. Where the present struggles are caused by a data stack from the past, Deep Lake emerges as a promising future for the AI data stack.
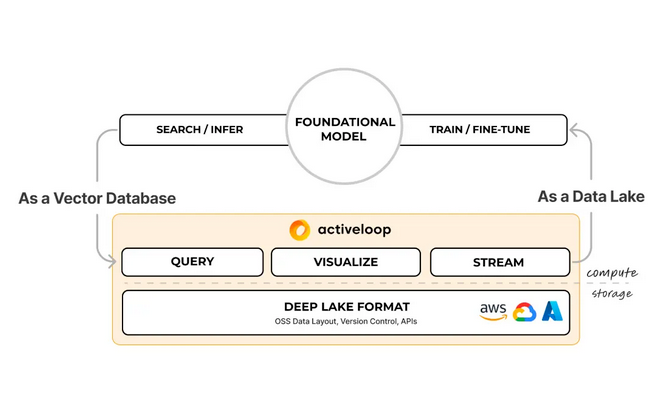
Activeloop, a company specializing in AI data infrastructure, recently announced its $11 million Series A funding round backed by Streamlined Ventures, Y Combinator, Samsung Next, Alumni Ventures, and Dispersion Capital. Activeloop's journey began in 2018 after founding CEO Davit Buniatyan experienced the hardships of leveraging large-scale unstructured datasets for AI. To address the challenges of managing large, complex, unstructured datasets for AI, the company started building Deep Lake, a vector database designed to accommodate all AI data.
Activeloop's current success is partly based on the groundbreaking contributions it has enabled. Buniatyan recalls that an early Activeloop customer required a model that could search through 80 million legal documents. As expected, Activeloop optimized and accelerated the model's training time from two months to a week. However, the significance of their achievement would not be evident for some time: Activeloop built a large language model when the Transformer was but a year old, way before OpenAI released the first iteration of the GPT models. With the advent of massive foundational multimodal models, Activeloop diligently works to align its flagship product Deep Lake with the needs of the modern ML development cycle and the multimodal AI data stack. It is hard to envision a company better suited to reflect on the past, present, and future of AI data.
Buniatyan cleverly sets the stage by expanding upon the current state of the AI data landscape, with all its complexities, unsolved issues, and foreshadowed challenges. Enterprises and organizations are sitting on massive collections of unexploited data of several types. At the same time, they are fully aware that a combination of retrieval augmentation (RAG) and fine-tuning is the ideal approach to obtain the best possible results, and that the 'data flywheel' enables an ideal in which training iterations are accelerated to nearly real-time speed since they take place using 'real-life' collected data. Fast training iterations are the best-kept secret of industry leaders such as Tesla, and the key behind groundbreaking text-to-image models DALL-E and Midjourney. Unfortunately, besides being complex and hard to build, the data flywheel is also (prohibitively) expensive. As a result, it remains out of reach for most enterprises and smaller organizations.
According to Buniatyan, many of these issues stem directly from "the ghost of 'AI data management’s past'", also known as the 2010s data stack. Surveys show that an important percentage of company data remains unused, and we have already examined some of the issues related to database management. However, there is also the issue that traditional and vector databases require to store data in the working memory to run queries at the expected speed, which introduces a further dilemma: organizations need to spend a lot of money to buy in-memory storage to retain performance, or they can opt for more budget-friendly alternatives at a speed cost. Then, even if we consider this issue solved, the current state of the AI stack has also inherited a noticeable gap between training and production environments. In practice, this translates to uneven data flow, since most engineers and data scientists hit by the ‘integration tax’ scramble to piece together a workflow made out of disjoint systems that will likely require resources spent in data translation across tools to make the workflow run. The resulting solutions are neither unified nor standardized, further complicating fast training iterations and making it unnecessarily complicated to scale local approaches to an entire organization.
Enter Deep Lake as a worthy contender for the future of AI data. Deep Lake is serverless, ensuring the independent operation of computing and storage components in the data stack. In addition to allowing for flexibility and scalability, it frees organizations from the overhead of physical server management, guarantees efficient resource allocation based on the data processing workload, and as a result, maximizes overall performance while keeping costs low. Multimodality is neatly handled by storing the various types of unstructured data in a unified tensor format allowing more accurate results across more diverse use cases. With fragmentation being the final piece in the AI data stack puzzle, Deep Lake simplifies AI development by being an all-in-one platform for data streaming, querying, and visualization, and effectively eliminating the need to piece together Frankenstein solutions from, at best, partially compatible tools.
Undoubtedly, Activeloop's Series A milestone is just the first step in the right direction. We are excited to see a future in which Deep Lake will undoubtedly deliver improved AI-native format data storage, live datasets resulting from the intersection between training and production data, and compound AI systems able to morph memory into models.