Adaptive ML secured $20M in seed funding to democratize LLM preference tuning
Adaptive ML is tackling the hurdles that keep enterprises and individuals from interacting with highly customized and user-personalized generative AI experiences. To this end, the company has secured $20M in seed funding to accelerate the development of its enterprise platform, Adaptive Engine.
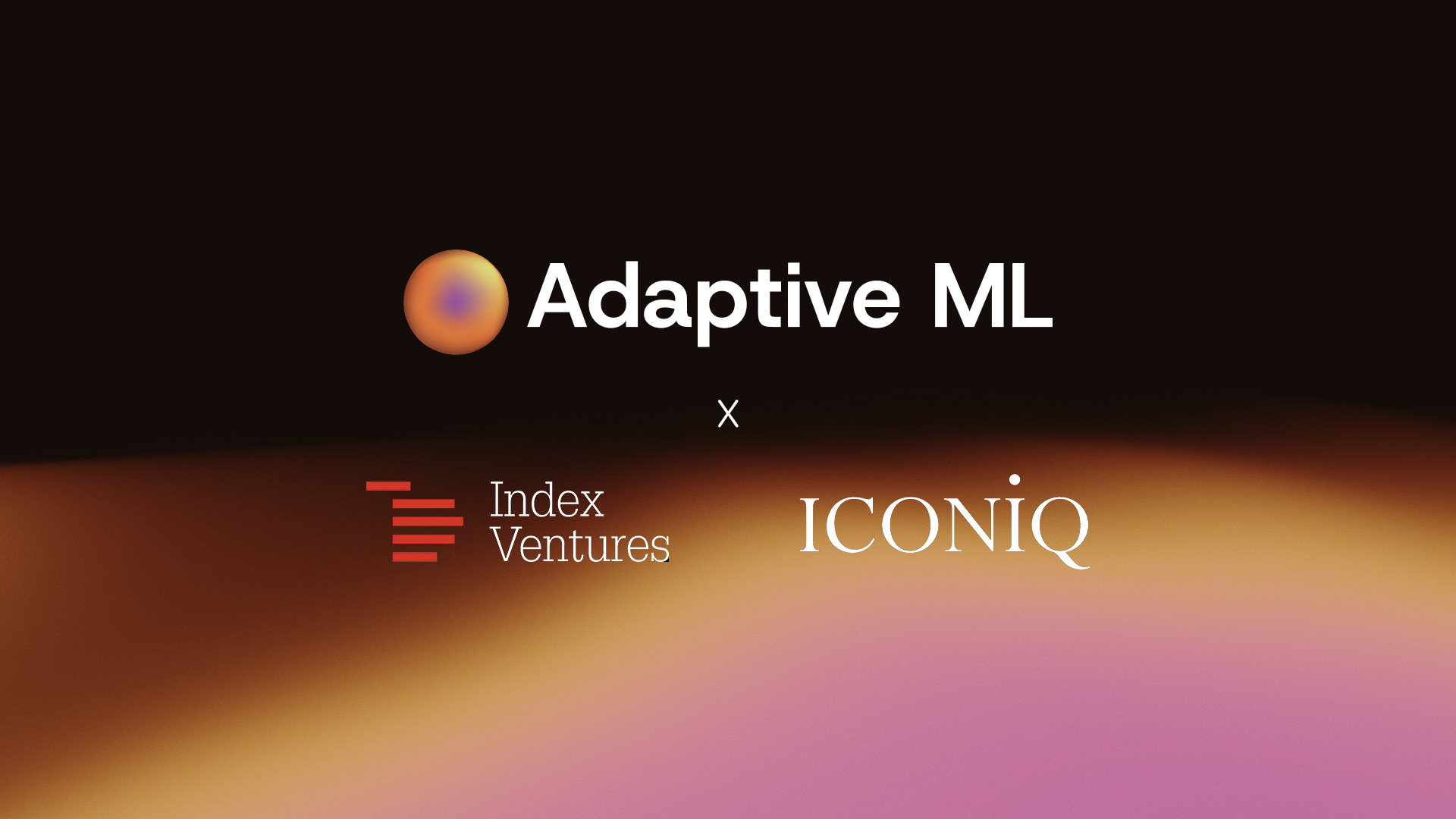
Adaptive ML has set it upon itself to end generic model deployments in enterprises and unchanging, impersonal individual model interactions. According to the startup, preference tuning is an effective solution for customization and adaptation to specific contexts and use cases, but is also out of reach for many enterprise and individual generative AI users. The most efficient preference tuning methods can be quite complicated to deploy. Moreover, they can get expensive, fast. Reinforcement learning from human feedback is undoubtedly a powerful method but relies on a massive workforce of human annotators creating equally substantial amounts of data non-stop. Contracts for such a workforce have been known to cost as much as the computing infrastructure required to train the model in the first place.
Thus, Adaptive ML's premise is that the first step towards true enterprise-level customization and individual per-user personalization is making preference tuning accessible to everyone. To this end, the startup has developed Adaptive Engine, its enterprise platform that empowers users to enhance their enterprise AI strategies by leveraging state-of-the-art preference-tuning methods that enable their models to learn directly from user interactions. This results in intuitive and engaging applications that improve user interactions and business outcomes.
Adaptive Engine is the startup's proposed solution to what they call "a three-body problem": the engineering complexities of preference tuning are solved with a specialized codebase, Adaptive Harmony, built from scratch with a focus on preference tuning; it avoids the costly implementation of RLHF by focusing on AI-provided feedback (RLAIF) and methods that can be implemented once the use case is in production; Finally, Adaptive Engine enables models to learn from their environments perpetually and with minimal human intervention but also features observability tools that ensure users that their models are working as expected.
Adaptive's mission has been accelerated by a successful $20 million seed funding round led by Index Ventures, with participation from ICONIQ Capital, Motier Ventures, Databricks Ventures, IRIS, HuggingFund by Factorial, and individual investors Xavier Niel, Olivier Pomel (Datadog), Dylan Patel (SemiAnalysis), and Tri Dao (FlashAttention). The funding, jointly with the founding members' contributions to training notable open-source models Falcon and BLOOM provides a solid foundation for Adaptive ML to pursue its mission of singular generative AI experiences.