Aligning Instruction Tasks Unlocks Large Language Models as Zero-Shot Relation Extractors
QA4RE is a framework that aligns RE with question answering (QA). It enables LLMs to outperform strong zero-shot baselines by a large margin. This work illustrates a promising way of adapting LLMs to challenging tasks by aligning these tasks with more common instruction-tuning tasks like QA.
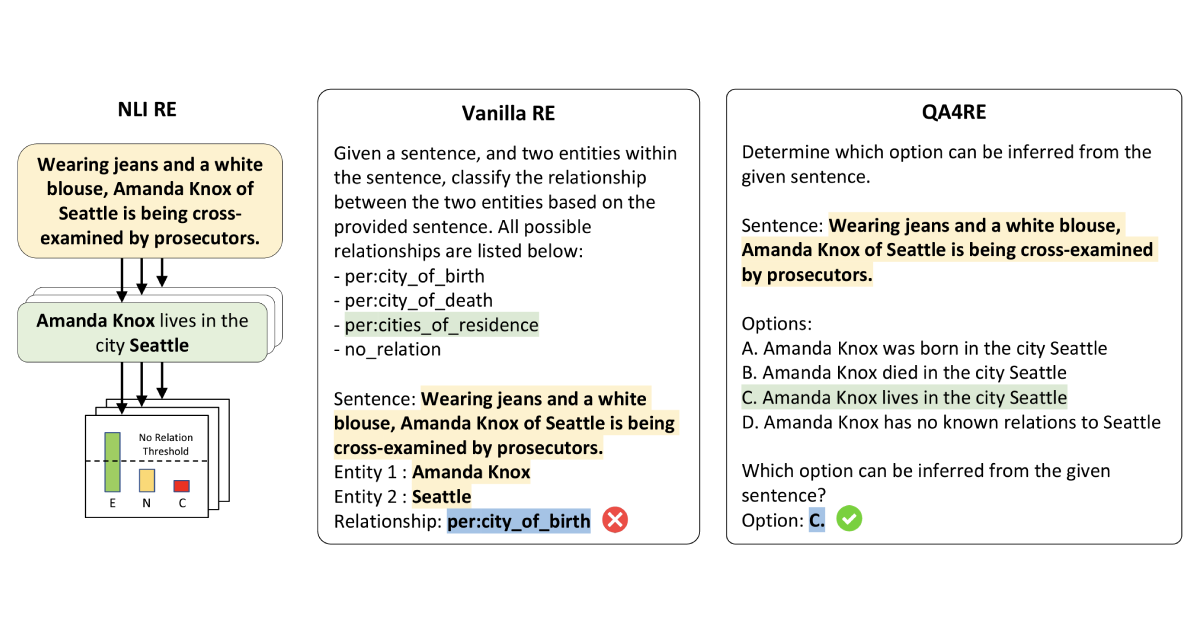
Kai Zhang, Bernal Jiménez Gutiérrez, Yu Su
Abstract
Recent work has shown that fine-tuning large language models (LLMs) on large-scale instruction-following datasets substantially improves their performance on a wide range of NLP tasks, especially in the zero-shot setting. However, even advanced instruction-tuned LLMs still fail to outperform small LMs on relation extraction (RE), a fundamental information extraction task. We hypothesize that instruction-tuning has been unable to elicit strong RE capabilities in LLMs due to RE's low incidence in instruction-tuning datasets, making up less than 1% of all tasks (Wang et al., 2022). To address this limitation, we propose QA4RE, a framework that aligns RE with question answering (QA), a predominant task in instruction-tuning datasets. Comprehensive zero-shot RE experiments over four datasets with two series of instruction-tuned LLMs (six LLMs in total) demonstrate that our QA4RE framework consistently improves LLM performance, strongly verifying our hypothesis and enabling LLMs to outperform strong zero-shot baselines by a large margin. Additionally, we provide thorough experiments and discussions to show the robustness, few-shot effectiveness, and strong transferability of our QA4RE framework. This work illustrates a promising way of adapting LLMs to challenging and underrepresented tasks by aligning these tasks with more common instruction-tuning tasks like QA.
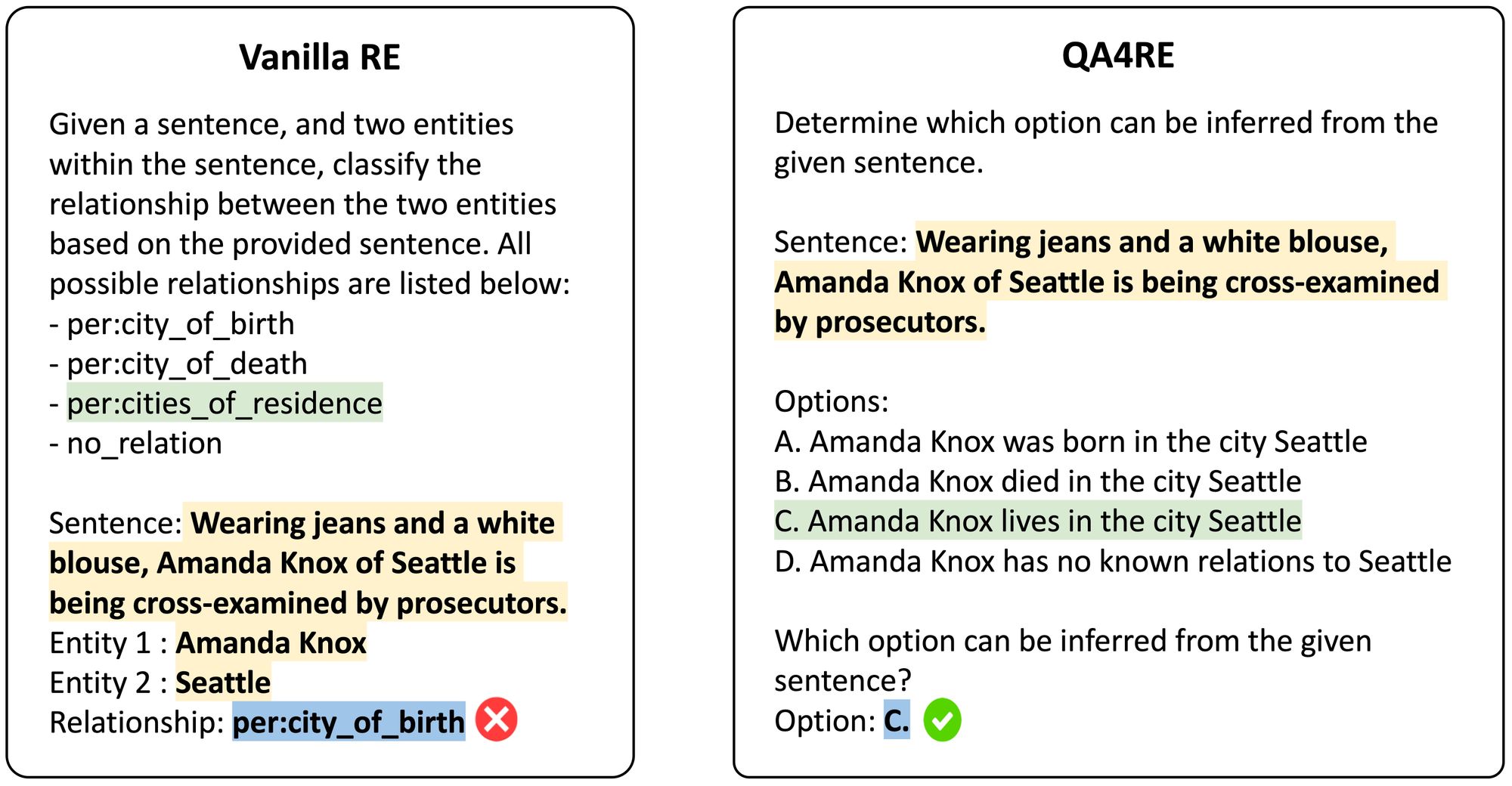
Results
QA4RE works on GPT-3.5 Series and FLAN-T5 Series, 6 LLMs in total
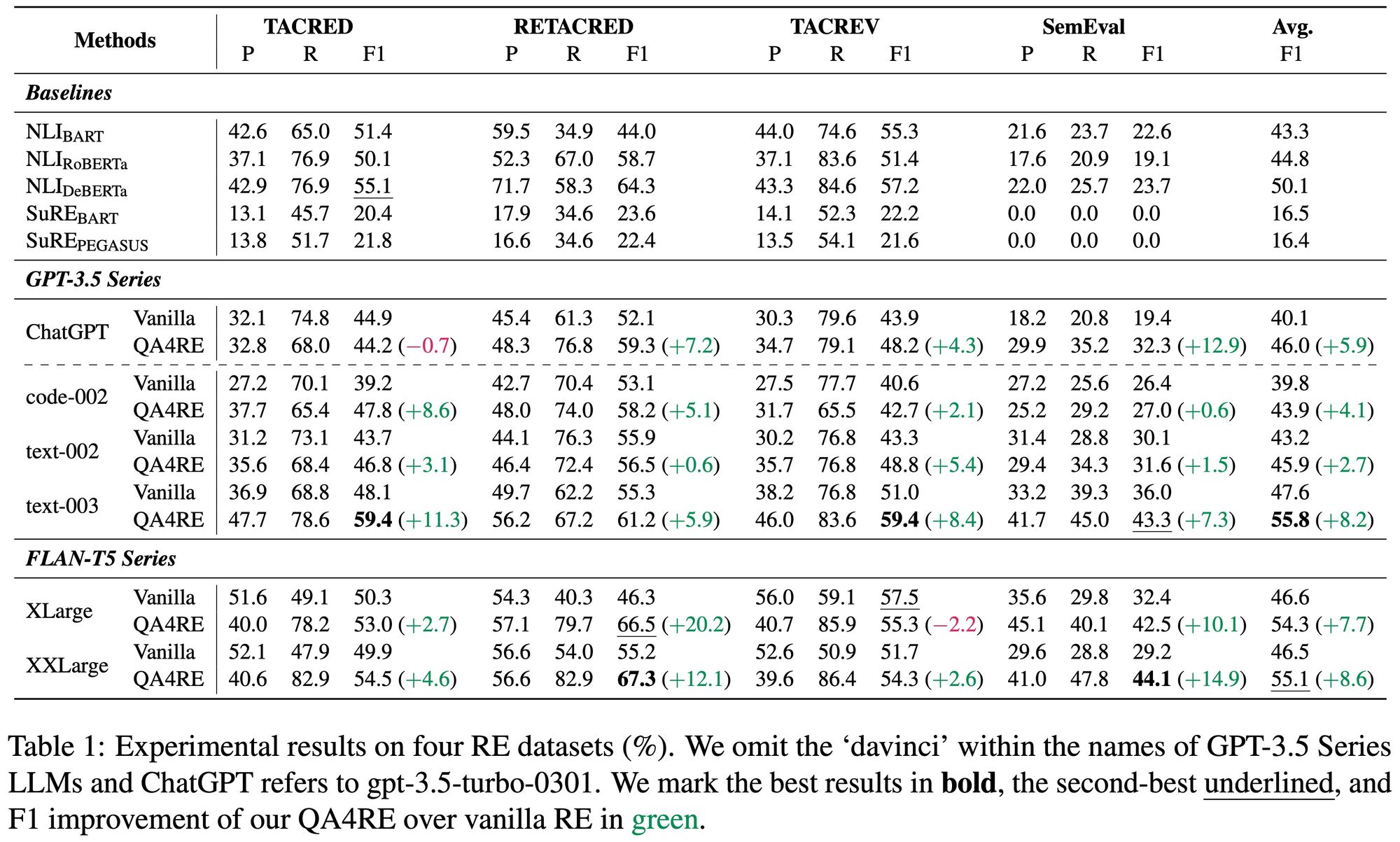
QA4RE works on smaller instruction-tuned models.
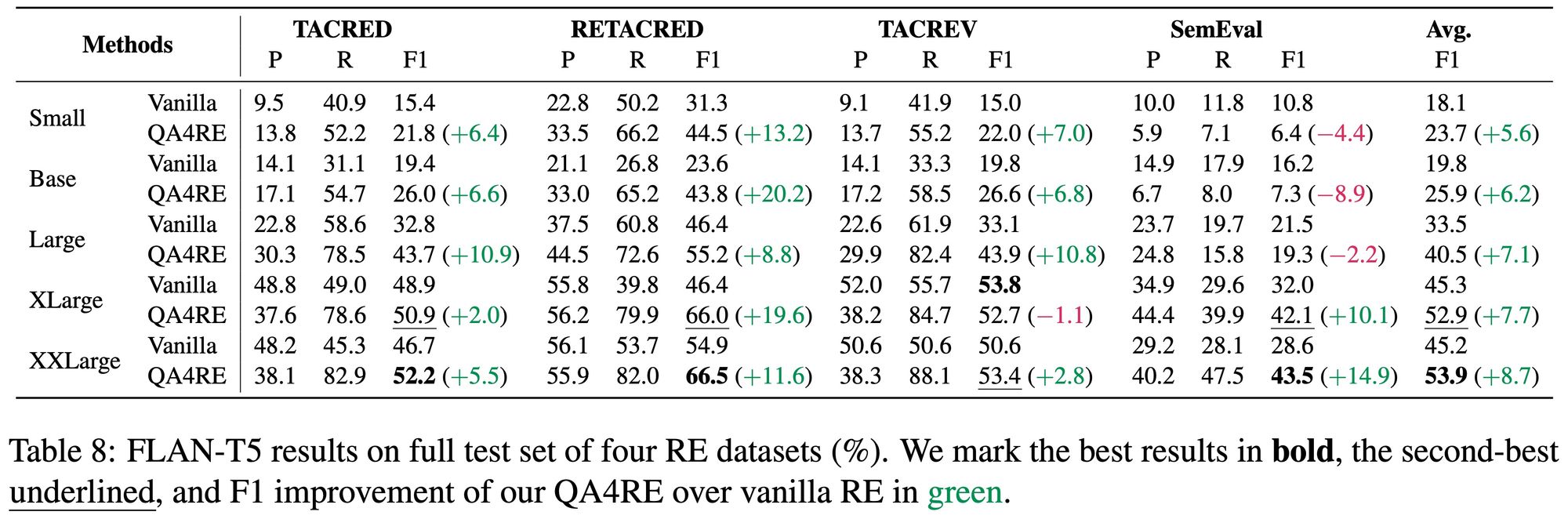