Arize Debuts Phoenix, the First Open Source Library for Evaluating Large Language Models
Arize AI, a leader in machine learning observability, has unveiled Phoenix, an open-source observability library designed for evaluating large language models (LLMs). Phoenix will enable data scientists to visualize complex LLM decision-making, monitor LLMs when they produce false or misleading results, and narrow in on fixes to improve outcomes. This
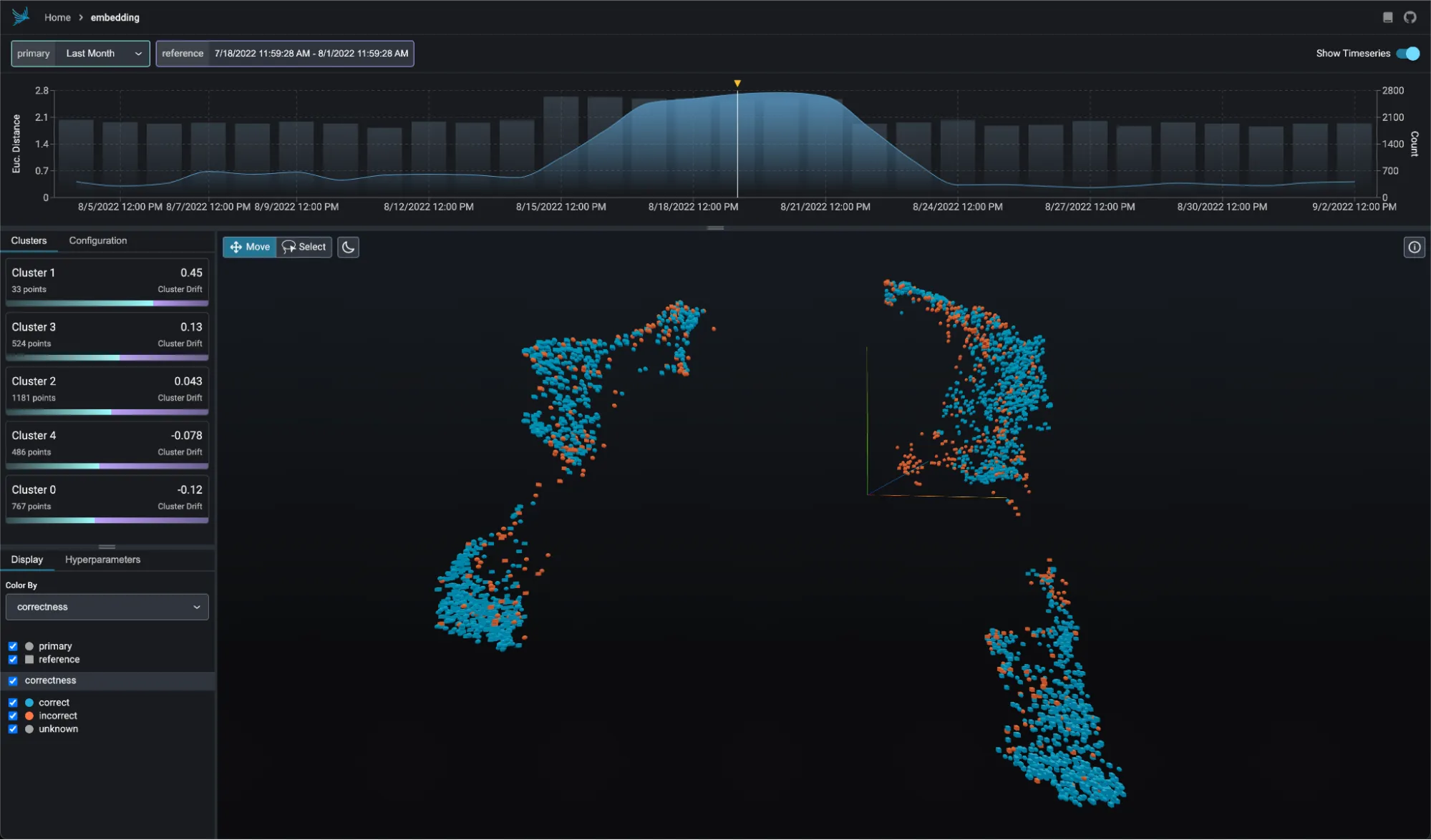
Arize AI, a leader in machine learning observability, has unveiled Phoenix, an open-source observability library designed for evaluating large language models (LLMs). Phoenix will enable data scientists to visualize complex LLM decision-making, monitor LLMs when they produce false or misleading results, and narrow in on fixes to improve outcomes. This development will allow businesses to better validate and manage risk for LLMs such as OpenAI’s GPT-4, Google’s Bard, and Anthropic’s Claude as they integrate into critical systems.
Generative AI is fueling a technical renaissance, with models such as GPT-4 exhibiting sparks of artificial general intelligence, and new breakthroughs and use cases emerging daily. However, most leading LLMs are black boxes with known issues around hallucination and problematic biases. Therefore, Phoenix's launch is timely, and it will enable data scientists to mitigate these issues.
The tool works easily with unstructured text and images, with embeddings and latent structure analysis as a core foundation of the toolset. Leveraging Phoenix, data scientists can troubleshoot tasks such as summarization or question/answering, detect anomalies, find clusters of issues to export for model improvement, surface model drift and multivariate drift, easily compare A/B datasets, map structured features onto embeddings for deeper insights, and monitor model performance and track down issues through exploratory data analysis.
Phoenix is instantiated by a simple import call in a Jupyter notebook and is built to interactively run on top of Pandas dataframes. According to Harrison Chase, Co-Founder of LangChain, "A huge barrier in getting LLMs and Generative Agents to be deployed into production is because of the lack of observability into these systems." Christopher Brown, CEO and Co-Founder of Decision Patterns, adds that Phoenix is a "much-appreciated advancement in model observability and production."
Arize AI's CEO and Co-Founder, Jason Lopatecki, affirms that despite calls to halt AI development, innovation will continue to accelerate. He states, "Phoenix is the first software designed to help data scientists understand how GPT-4 and LLMs think, monitor their responses, and fix the inevitable issues as they arise."
Arize AI's automated model monitoring and observability platform allows ML teams to quickly detect issues when they emerge, troubleshoot why they happened, and improve overall model performance across both structured and unstructured data. Arize is a remote first company with headquarters in Berkeley, CA.