Dear readers,
I hope you’re all doing great! So, I’d like to commemorate the 50th issue of the digest to a few things that are important to me :)
#1
This Sunday, July 24, I’ll be running a half-marathon on The San Francisco Marathon. In this race, I am participating in a charity fundraising for the KOLO fund that helps the Ukrainian army. I invite everyone to join, to help me raise donations. You can donate any sum you want, and you can also subscribe.
#2
The Data Phoenix is excited to announce the launch of our yearly survey — Machine Learning & Data Science Survey 2022 — among those who’re engaged in Machine Learning, Computer Vision, Natural Language Processing, Data Science, and other aspects of Artificial Intelligence.
I invite all our readers to answer some questions about your expertise, skills, and toolsets. You’ll help us figure out what’s going on in the industry in 2022. Of course, we’ll share the results later!
Слава Україні!
Best regards,
Dmitry Spodarets
Chief Editor of Data Phoenix
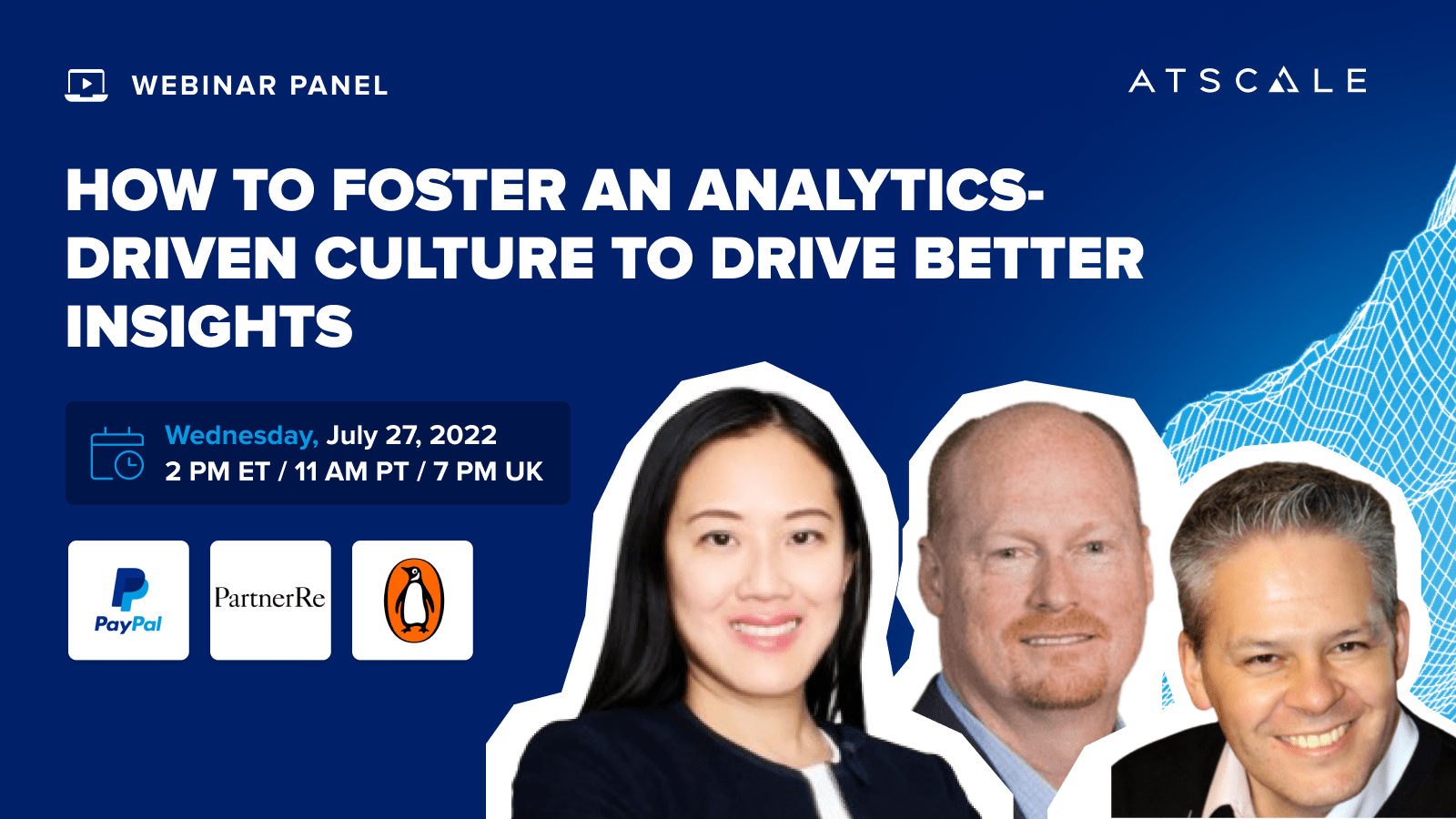
Get practical advice from Data & Analytics Leaders from PayPal, Penguin Random House, & PartnerRe to learn about fostering an analytics-driven culture to drive better insights.
ARTICLES
How to Test ML Models in the Real World
In this article, you will learn about the evaluation methods that can allow you to efficiently test ML models in the real world, to convince your leadership that they add value to the business.
Deploying Transformers on the Apple Neural Engine
In this article, Apple shares the principles behind the Apple Neural Engine (ANE) to provide generalizable guidance to developers on optimizing their models for ANE execution.
Automated Testing in Machine Learning Projects [Best Practices for MLOps]
Automated testing in machine learning is a relatively new topic. This article explains all the ins and outs of it so that you could design, build, and deliver complicated ML systems more easily.
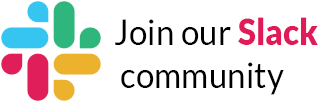
DeepETA: How Uber Predicts Arrival Times Using Deep Learning
At Uber, magical customer experiences depend on accurate arrival time predictions (ETAs). Learn how Uber developed a low-latency deep neural network architecture for global ETA prediction.
Systematic Way to Extract Features From Image Data
In this post, you’ll learn how to reduce the dimension of a picture to fight the curse of dimensionality, to be able to extract features that are useful for modeling.
Best Practices for Deploying Language Models
Cohere, OpenAI, and AI21 Labs have developed a preliminary set of best practices applicable to any organization developing or deploying large language models. Here’s the list of them.
Session-Based Recommender Systems with Word2Vec
In this post, you’ll discover a new approach for applying Python to train a basic recommender system with Word2Vec on browser session data. Check it out!
Super-Resolution Generative Adversarial Networks (SRGAN)
SRGANs achieve better image super-resolution results by combining the traditional GAN elements with recipes intended to elevate visual performance. Learn more about them in this guide!
PAPERS
Mask DINO: Towards A Unified Transformer-based Framework for Object Detection and Segmentation
Mask DINO is a unified object detection and segmentation framework with a mask prediction branch which supports all image segmentation tasks (instance, panoptic, and semantic).
Evaluation-oriented Knowledge Distillation for Deep Face Recognition
In this paper, the authors propose a novel method for deep face recognition to reduce the performance gap between the teacher and student models during training.
Separable Self-attention for Mobile Vision Transformers
This paper introduces a separable self-attention method with linear complexity that uses element-wise operations for computing self-attention for resource-constrained devices.
MotionCNN: A Strong Baseline for Motion Prediction in Autonomous Driving
In this paper, the authors present a baseline for multimodal motion prediction based on CNNs. It achieves competitive performance compared to the state-of-the-art methods.
CVNets: High Performance Library for Computer Vision
CVNets is a high-performance open-source library for training deep neural networks for visual recognition tasks, including classification, detection, and segmentation.
PROJECTS
Neural 3D Reconstruction in the Wild
The project presents a new method for accurate surface reconstruction from Internet photo collections in varying illumination, by using a hybrid voxel- and surface-guided sampling technique.
StylizedNeRF: Consistent 3D Scene Stylization as Stylized NeRF via 2D-3D Mutual Learning
StylizedNeRF combines a 2D image stylization network and NeRF to fuse the stylization ability of 2D stylization network with the 3D consistency of NeRF.
COURSES
MLOps Zoomcamp
During the MLOps Zoomcamp course, you’ll explore and learn the practical aspects of productionizing ML services, from collecting requirements to model deployment and monitoring.
Python for Machine Learning (7-day mini-course)
In this crash course, you will discover how concise a Python code can be, and how much the functions from its libraries can do in relation to your machine learning tasks.
CODE & TOOLS
- PaddleSpeech is an open-source toolkit on PaddlePaddle platform for a variety of critical tasks in speech and audio, with the state-of-art and influential models. Have a look on GitHub!
- PyGOD is a Python library for graph outlier detection that includes more than 10 latest graph-based detection algorithms, such as DOMINANT (SDM'19) and GUIDE (BigData'21).
Comments