Researchers at Google and DeepMind have made the groundbreaking discovery of 2.2 million new inorganic crystals with the assistance of Graph Networks for Materials Exploration (GNoME). GNoMe is a deep learning tool that accelerates the discovery of new materials by predicting their stability. In this case, 380,000 of the discovered crystals were classified as the most stable, making them candidates for experimental synthesis. Some of the materials in the list may have the potential to push technological development forward by becoming superconductors, powering supercomputers, or being at the core of a new generation of more efficient rechargeable batteries.
A recently published paper in Nature introduces GNoME and its discovery of over 2.2 million stable structures by building on the previous knowledge of 48,000 stable structures identified in continued studies based on traditional and computational methods. Moreover, the paper also states that 736 of the predicted structures have already been independently experimentally realized, with potentially more underway after a group of researchers at the Lawrence Berkeley National Laboratory, in partnership with Google DeepMind, published a second paper in Nature where they explore the possibility of leveraging AI to create an autonomous laboratory that can accelerate the experimental synthesis of new materials.
Until recently, discovering stable structures by manual methods, such as tweaking known crystals or experimenting with new combinations of elements, has uncovered 20,000 computationally stable materials out of the 200,000 entries in the Inorganic Crystal Structure Database (ICSD). This has proven to be a costly and lagging strategy that relies upon trial-and-error experimentation based on chemical intuition. Computational approaches to materials prediction and discovery, such as the Materials Project, have improved the outlook by boosting the number of known viable materials to 48,000. However, even these approaches have hit a fundamental limit in their capacity to predict experimentally feasible structures.
According to the DeepMind team's calculations, GNoME's groundbreaking 2.2 million predictions amount to approximately 800 years' worth of knowledge. GNoME's predictions include 52,000 new layered compounds similar to graphene that could accelerate the development of new superconductors and 528 potential lithium ion conductors that could revolutionize how rechargeable batteries are built. The list containing the 380,000 most promising predictions that could result in viable materials for downstream applications has been released to foster new research.
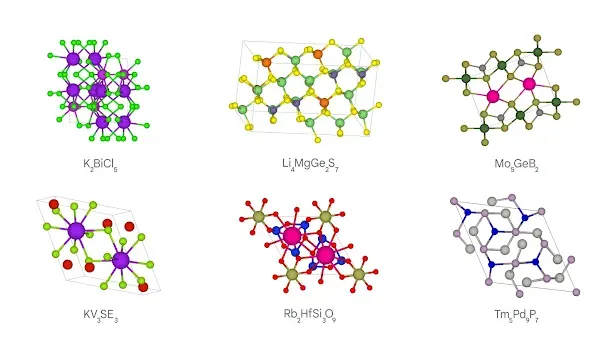
The 380,000 candidates that are stable by current scientific standards lie below the convex hull of previous discoveries, which means that GNoME has not only accelerated the discovery of new potentially viable materials but has helped set an entirely new standard for materials stability. GNoME's achievements are nothing short of extraordinary, and even more so once one considers this is the second time this year that the researchers at DeepMind have proven the potential of deep learning for accelerating prediction and discovery in fields dominated by time-consuming and expensive manual methods, such as protein structure prediction. It is thrilling to think that with this, the research teams at DeepMind and their collaborators may have brought us one step closer to unlocking a future that holds all sorts of medical, technological, and sustainability breakthroughs.
Comments