eDiffi: Text-to-Image Diffusion Models with Ensemble of Expert Denoisers
eDiff-I is the next generation of generative AI content creation tool that offers unprecedented text-to-image fusion, instant style transfer, and intuitive word-painting capabilities. This diffusion model for image synthesis from text is based on T5 text inlays, CLIP image inlays, and CLIP text inlays. This approach generates photorealistic images that
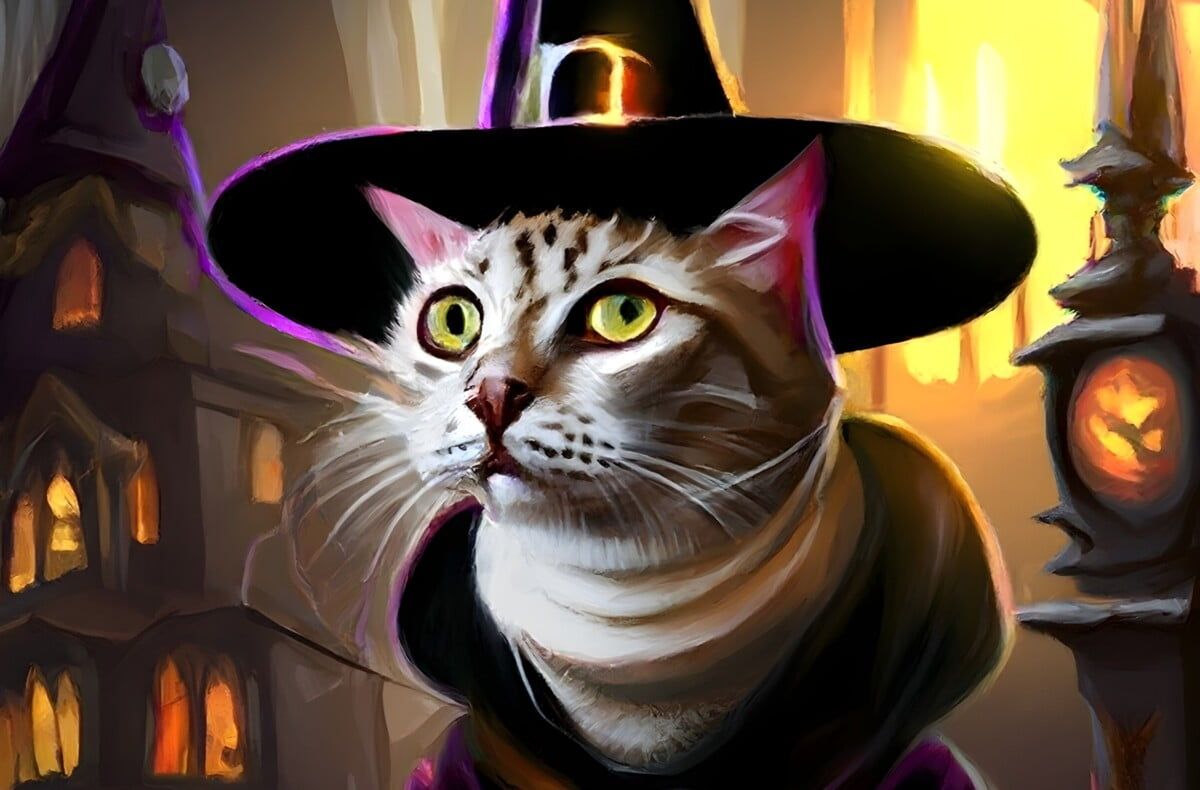
eDiff-I is the next generation of generative AI content creation tool that offers unprecedented text-to-image fusion, instant style transfer, and intuitive word-painting capabilities.
This diffusion model for image synthesis from text is based on T5 text inlays, CLIP image inlays, and CLIP text inlays. This approach generates photorealistic images that match any input text query.
The eDiff-I consists of a cascade of three diffusion models. The first is a base model that can synthesize samples at 64x64 resolution. Then there are two super-resolution stacks that can incrementally increase the image resolution to 256x256 and 1024x1024, respectively. The models take the input caption and compute T5 XXL embedding and text embedding. CLIP image encodings, which are computed from the reference image, can also be used. The image embeddings can serve as a style vector, and then they are fed into cascading diffusion models which gradually generate images at 1024x1024 resolution.
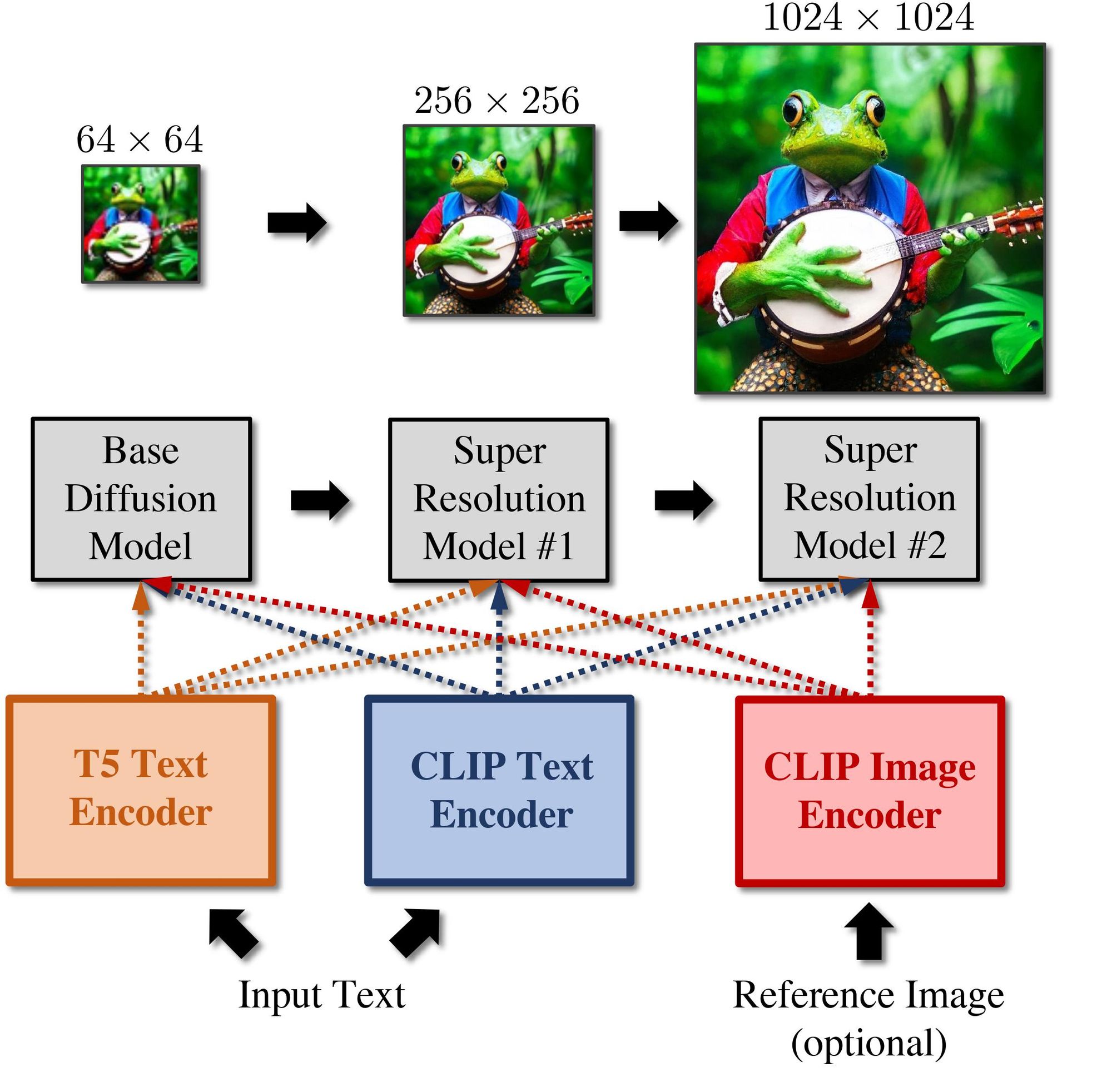
In addition to synthesizing text into images, the eDiff-I model has two additional features - style transfer, which allows you to control the style of the generated pattern using a reference image, and "Paint with words," an application in which the user can generate images by drawing segmentation maps on the canvas, which is very useful for creating the desired image.
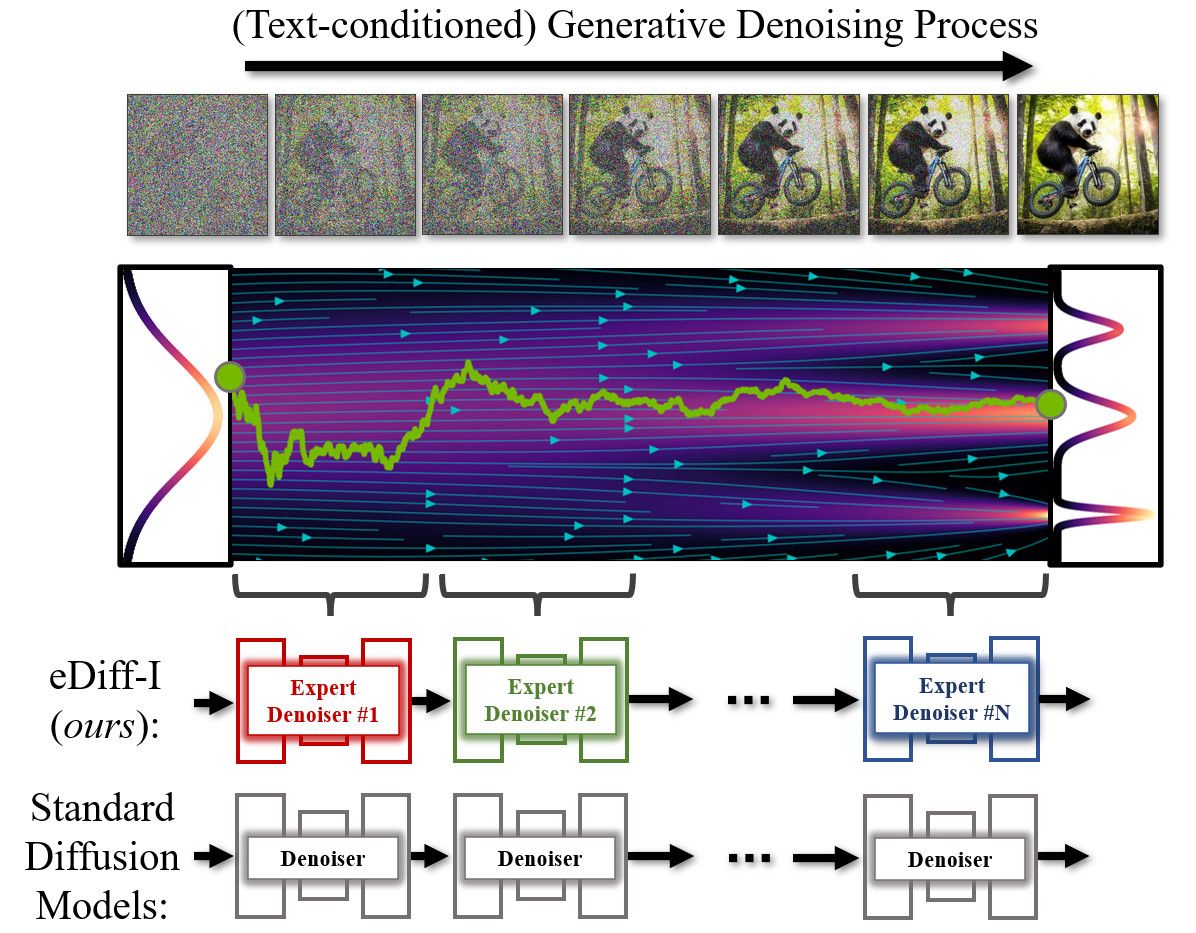
In diffusion models, image synthesis occurs through an iterative denoising process that gradually generates images from random noise. The model starts with complete random noise, which is then gradually subjected to a multi-step denoising process to eventually produce an image. In conventional diffusion model training, one model is trained to denoise the entire noise distribution. But in the eDiff-I system, instead, an ensemble of expert denoising models is trained that specialize in denoising in different intervals of the generative process. The use of such expert denoisseurs leads to improved synthesis capabilities.
- Project - https://deepimagination.cc/eDiffi/
- Paper - https://arxiv.org/abs/2211.01324
Meanwhile, the Musika music generation system, which can be trained on hundreds of hours of music using a single consumer GPU, and which can generate music of arbitrary length much faster than in real time on a consumer CPU, is gaining more and more admiration from users.