Imperial College London and DeepMind are researching embodied agents that learn more efficiently
Researchers from Imperial College London and Google DeepMind have developed DAAG, a novel AI framework that combines language and vision models to enhance embodied agents' learning efficiency and adaptability, addressing the challenge of limited training data in physical environments.
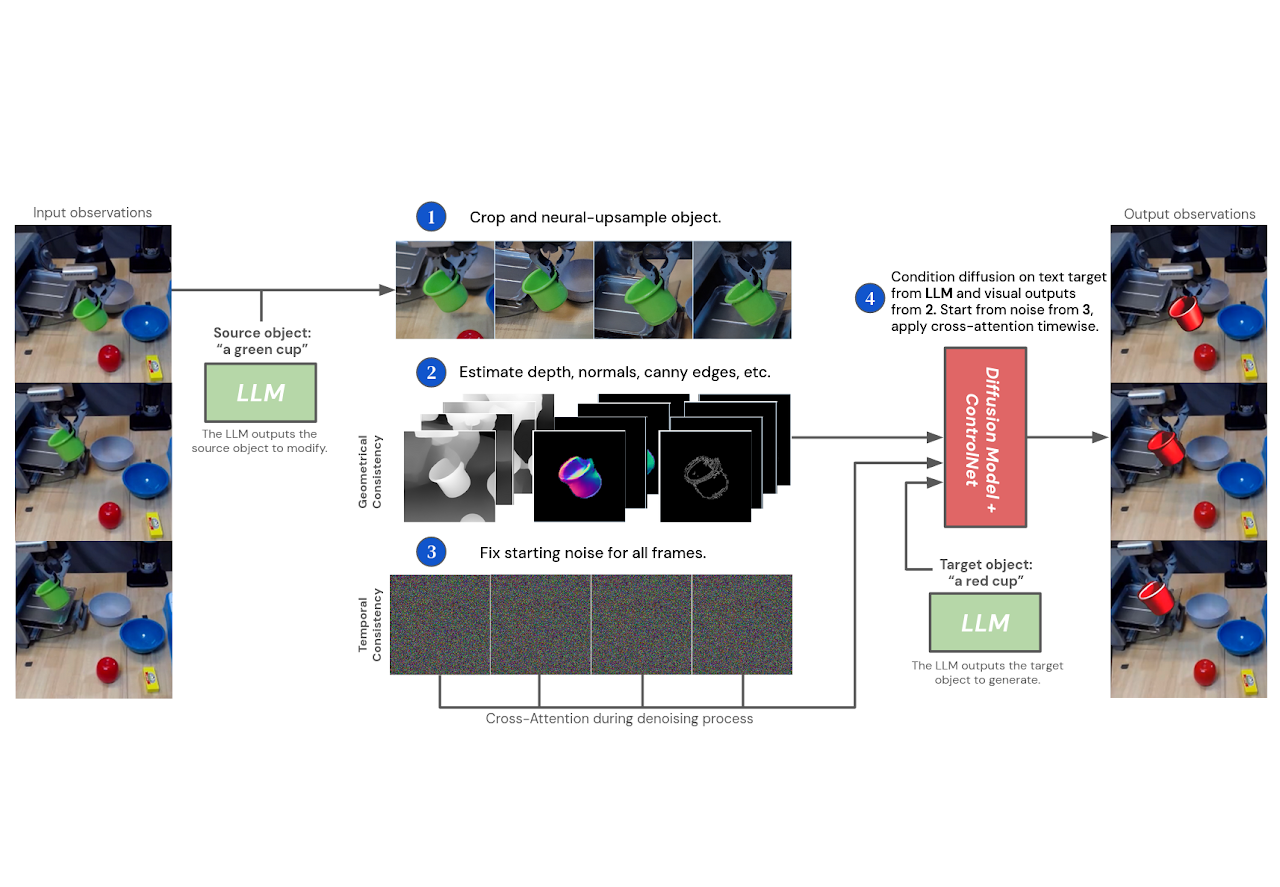
Researchers from Imperial College London and Google DeepMind have recently introduced a new agent framework called Diffusion Augmented Agents (DAAG), addressing a major challenge in embodied AI: the scarcity of training data. DAAG combines large language models (LLMs), vision language models (VLMs), and diffusion models in a framework that enables agents to become more efficient and adaptable by learning autonomously by reusing previous episodes stored in the framework's offline lifelong buffer, which stores all the agent's past experiences.
The process by which agents repurpose past episodes to learn new information is called Hindsight Experience Augmentation (HEA). With HEA, agents are first given a text-based instruction, which the LLM analyzes into subgoals. Then, given an episode of experience, the VLM labels it with the subgoals that the agent could have reached. If one of these subgoals matches the subgoal from the task at hand, it is stored in the task-specific buffer.
However, suppose the episode does not exactly match any of the subgoals in the ongoing task. In that case, the framework's LLM is asked if the episode would satisfy one of the subgoals if an object in the episode were modified, i.e. matching “The red cube is stacked on the blue cube” with “The green cube is stacked on the blue cube” by swapping red cube with green cube. If some such modification is possible, the LLM queries the diffusion model so it modifies the original observation to reflect the needed change. This process enables the agent to reuse as much data as possible and to synthetically increase the number of previous experiences it can take as starting points for new tasks.
The authors performed benchmarking tests over simulated environments to find that DAAG performed significantly better than traditional reinforcement learning systems. Agents using this framework achieved goals more quickly, learned without explicit rewards, and efficiently transferred knowledge between tasks. These are all necessary tasks in developing more efficient and adaptable agents. In particular, the authors believe their work suggests "promising directions for overcoming data scarcity in robot learning and developing more generally capable agents."