Meshy has launched Meshy-4, a model with improved 3D-generation capabilities
Meshy has launched Meshy-4, a major update to their AI-powered 3D model generation platform. Meshy-4 features improved geometry, a new Text to 3D workflow, a retry feature, and model selection options, and aims to enhance the quality and usability of AI-generated 3D assets.
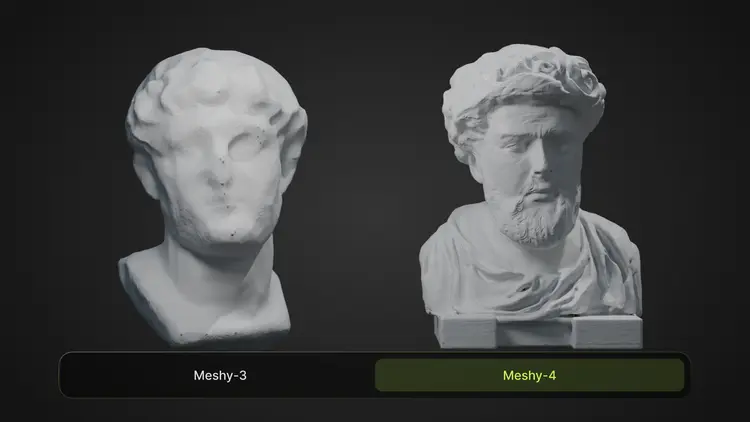
3D model generation is quickly becoming the next frontier for generative AI. For those players with a stake in the field, 3D model generations have become a matter of delivering swift, accurate, and detailed results suited for professional applications from visuals for artistic projects to world-building for game development and augmented reality. To gain an advantage in the 3D model generation race, Meshy recently announced the release of its foundation model for text-to-3D and image-to-3D generation, Meshy-4.
Meshy has worked on improving the generation algorithm powering Meshy-4, resulting in 3D models that boast improved geometry quality, as can be seen in models that feature clean and detailed hard surfaces, which are no longer afflicted by the bumps and dents appearing in the outputs of previous models. To showcase Meshy-4's capabilities, Meshy has updated its Discover page to reveal the untextured version of any artifact when users hover over it. By showcasing the mesh without textures, users can better appreciate Meshy-4's capabilities to deliver high-quality hard surfaces and intricate details.
With a new Meshy-4 text-to-3D workflow, Meshy addressed the reports from users experiencing a disconnect in the generation stages, with the Refined stage delivering outputs that significantly departed from the Coarse stage. The new Modeling and Texturing stages ensure that the first stage generates meshes that work as a foundation for the second one, thus guaranteeing high-quality and coherent generations. The separation between both processes will also pave the way for future edition capabilities. With this release, Meshy has also introduced the possibility to retry a generation without spending more credits when the previewed output does not match the user's expectations and to pick the model that will perform a given task.