Abstract
We present 3DiM (pronounced "three-dim"), a diffusion model for 3D novel view synthesis from as few as a single image. The core of 3DiM is an image-to-image diffusion model -- 3DiM takes a single reference view and a relative pose as input, and generates a novel view via diffusion. 3DiM can then generate a full 3D consistent scene following our novel stochastic conditioning sampler. The output frames of the scene are generated autoregressively. During the reverse diffusion process of each individual frame, we select a random conditioning frame from the set of previous frames at each denoising step. We demonstrate that stochastic conditioning yields much more 3D consistent results compared to the naïve sampling process which only conditions on a single previous frame. We compare 3DiMs to prior work on the SRN ShapeNet dataset, demonstrating that 3DiM's generated videos from a single view achieve much higher fidelity while being approximately 3D consistent. We also introduce a new evaluation methodology, 3D consistency scoring, to measure the 3D consistency of a generated object by training a neural field on the model's output views. 3DiMs are geometry free, do not rely on hyper-networks or test-time optimization for novel view synthesis, and allow a single model to easily scale to a large number of scenes.
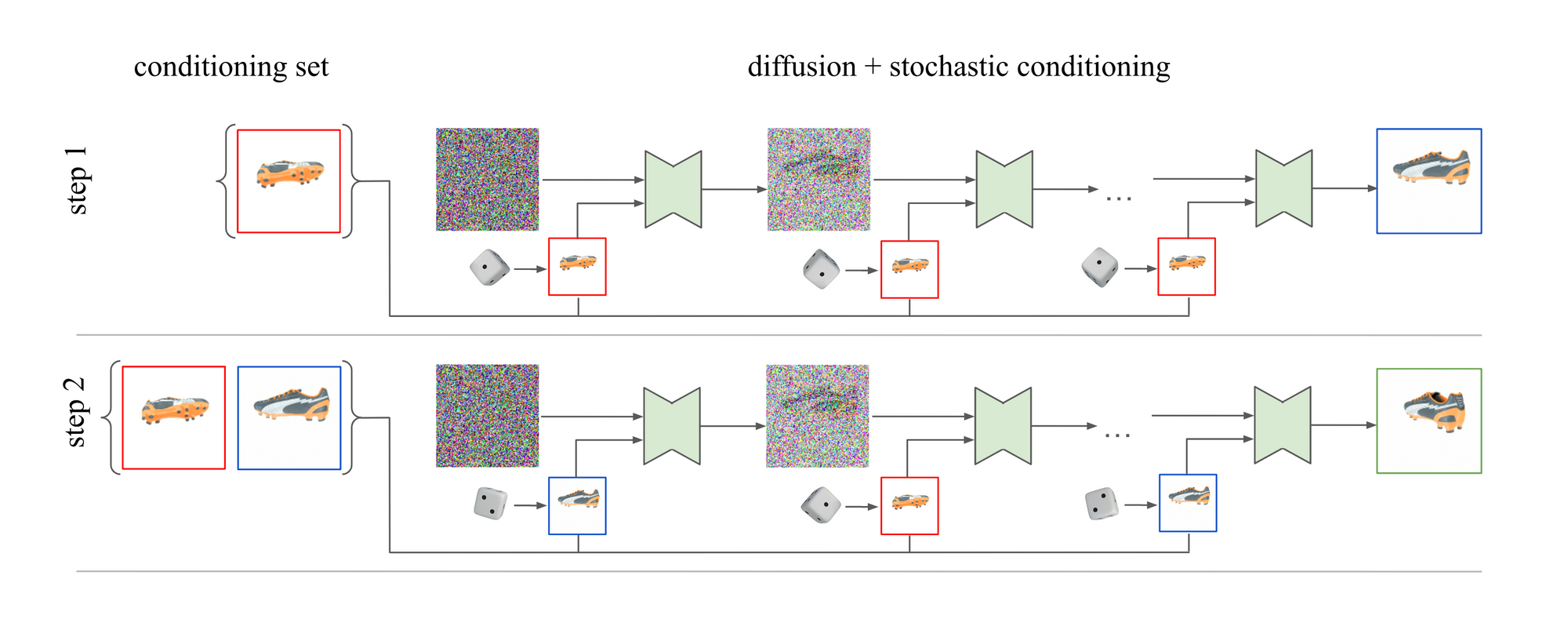
Comments