Xiangtai Li1, Haobo Yuan1, Wei Li1, Henghui Ding1, Size Wu1, Wenwei Zhang1,2,
Yining Li2, Kai Chen2, Chen Change Loy1
1S-Lab, Nanyang Technological University, 2Shanghai Artificial Intelligence Laboratory
Abstract
In this work, we address various segmentation tasks, each traditionally tackled by distinct or partially unified models. We propose OMG-Seg, One Model that is Good enough to efficiently and effectively handle all the segmentation tasks, including image semantic, instance, and panoptic segmentation, as well as their video counterparts, open vocabulary settings, prompt-driven, interactive segmentation like SAM, and video object segmentation. To our knowledge, this is the first model to fill all these tasks in one model and achieve good enough performance.
We show that OMG-Seg, a transformer-based encoder-decoder architecture with task-specific queries and outputs, can support over ten distinct segmentation tasks and yet significantly reduce computational and parameter overhead across various tasks and datasets. We rigorously evaluate the inter-task influences and correlations during co-training. Both the code and models will be publicly available.
Model Scope Comparison
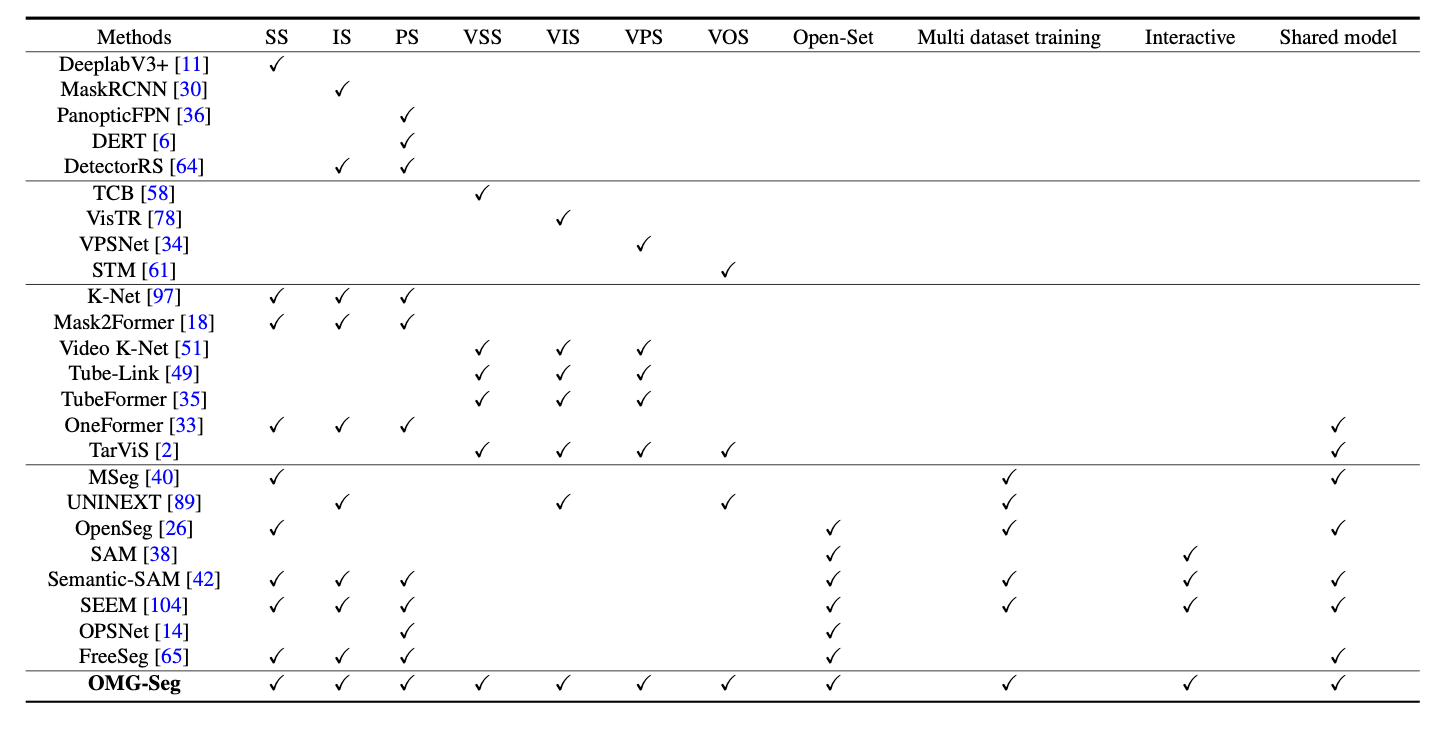
We include several representative methods here. Our proposed OMG-Seg can perform various segmentation tasks in one model. SS: Semantic Segmentation. IS: Instance Segmentation. PS: Panoptic Segmentation. VSS: Video Semantic Segmentation. VIS: Video Instance Segmentation. VPS: Video Panoptic Segmentation. VOS: Video Object Segmentation. Open-Set: Open-Vocabulary Segmentation.
Video
Method: OMG-Seg
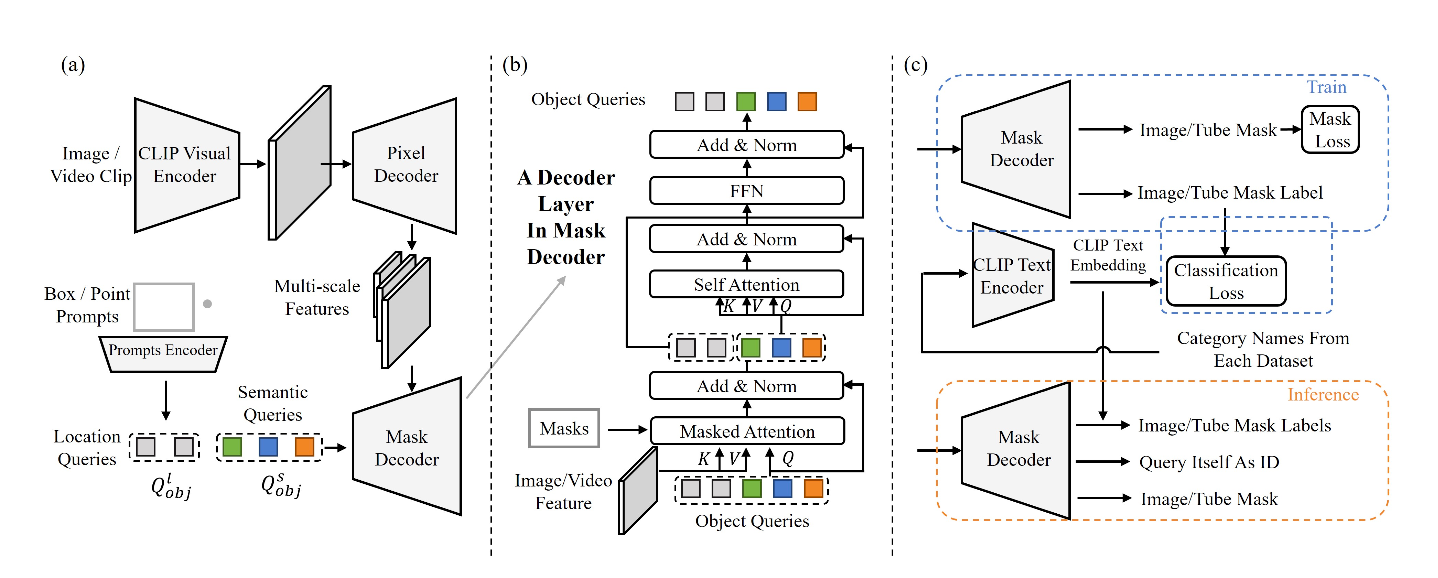
(a), OMG-Seg follows the architecture of Mask2Former, containing a backbone (CLIP Visual Encoder), a pixel decoder, and a mask decoder. The different parts are a shared mask decoder for both image and video segmentation and a visual prompt encoder. We use two types of mask queries, i.e., semantic queries, for instance/semantic masks or mask tubes, and location queries that encode box or point prompts. (b), One decoder layer in the Mask Decoder. The location queries skip the self-attention operation as they are only conditioned on the image content and the location prompts. (c), The forward pass of OMG-Seg in training and inference. We use CLIP's text encoder to represent category names and classify masks by calculating cosine similarity between mask features and text embeddings
Comments