Abstract
Dynamic radiance field reconstruction methods aim to model the time-varying structure and appearance of a dynamic scene. Existing methods, however, assume that accurate camera poses can be reliably estimated by Structure from Motion (SfM) algorithms. These methods, thus, are unreliable as SfM algorithms often fail or produce erroneous poses on challenging videos with highly dynamic objects, poorly textured surfaces, and rotating camera motion. We address this robustness issue by jointly estimating the static and dynamic radiance fields along with the camera parameters (poses and focal length). We demonstrate the robustness of our approach via extensive quantitative and qualitative experiments. Our results show favorable performance over the state-of-the-art dynamic view synthesis methods.
RoDynRF
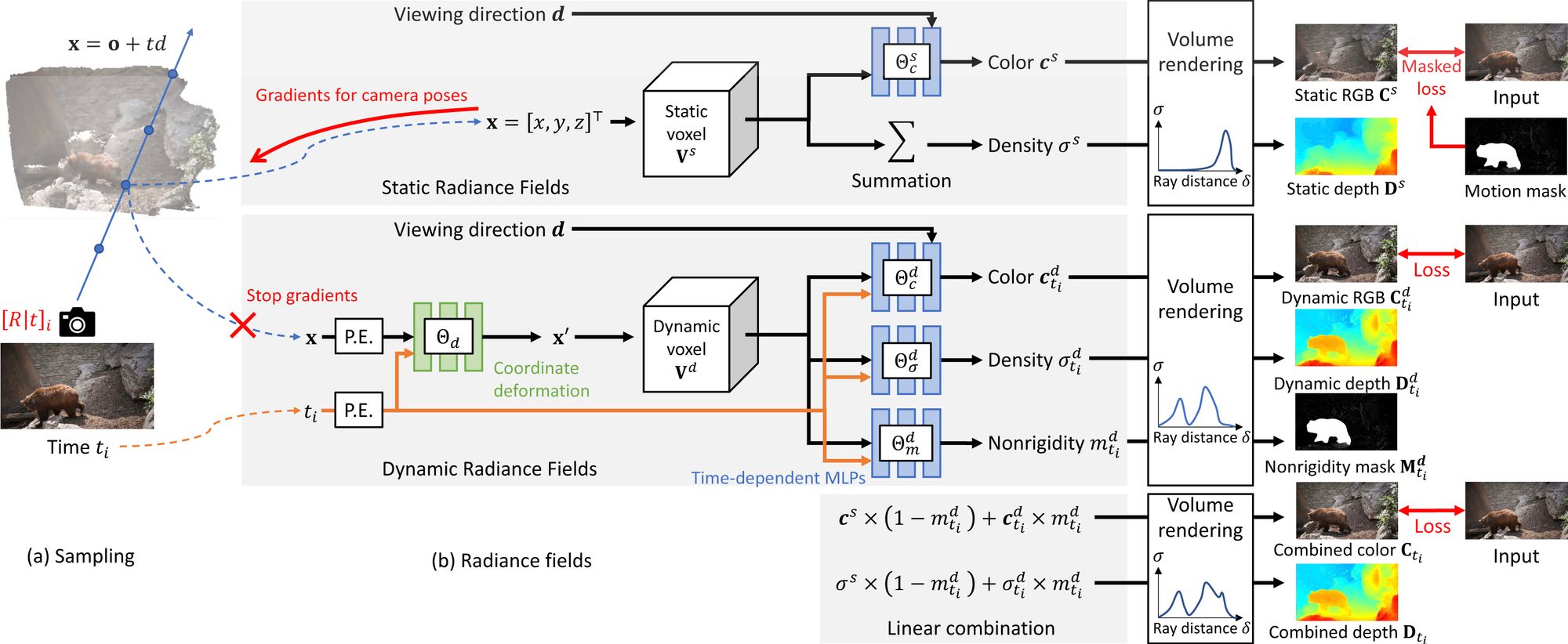
Comments