The 2023 State of AI Report is out
The 2023 State of AI Report has just been released, containing an in-depth analysis of the current situation in the field spanning from research and industry to politics and safety. The report also features the team's prediction for the most exciting developments to be seen in the following year.
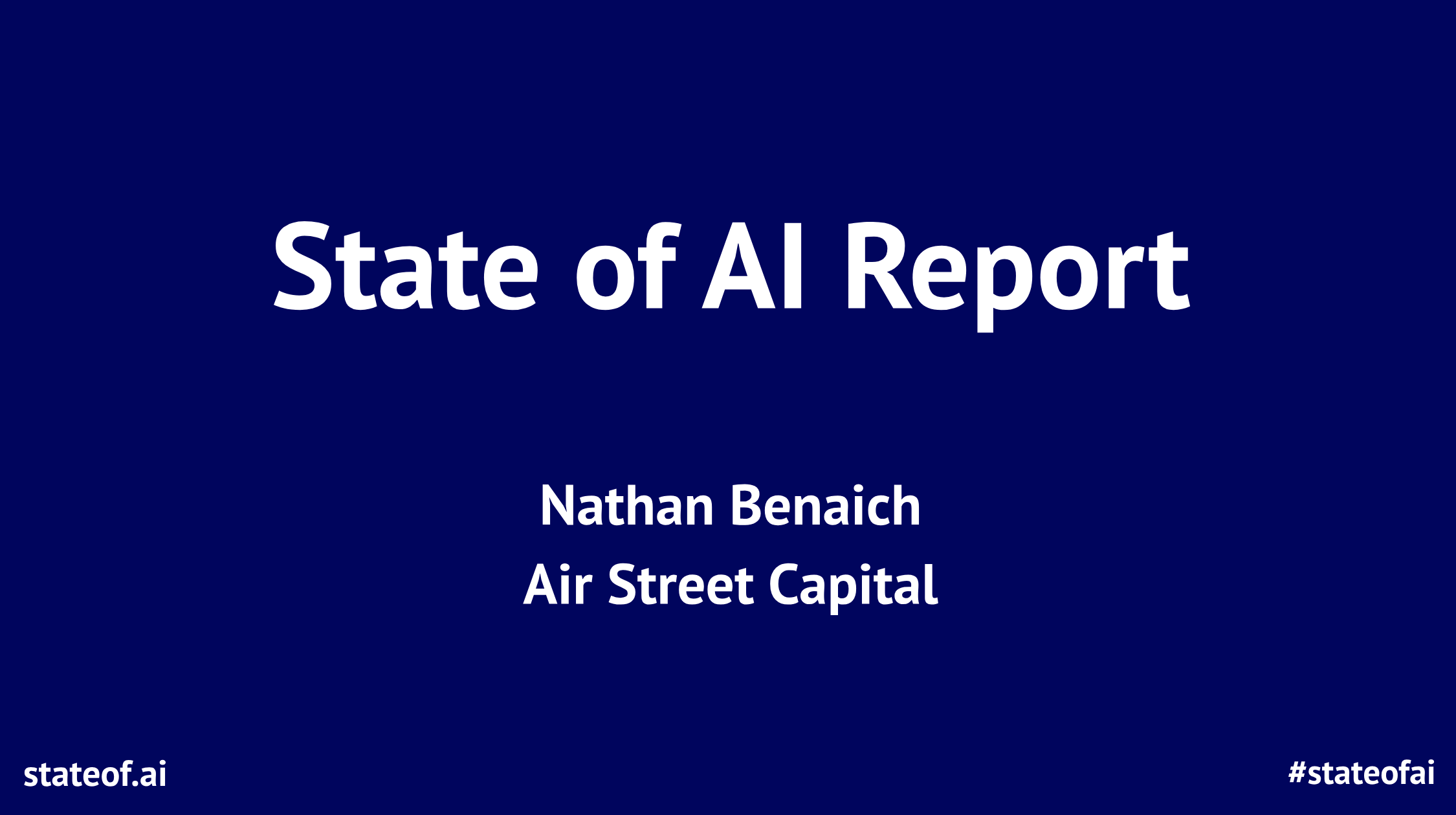
The sixth edition of the State of AI Report just came out bearing some fascinating news and confirming our long-held suspicions. Authored by Nathan Benaich and a team from Air Street Capital, the report goes deep into topics on AI research, industry, politics, safety, and the team's predictions for the most exciting developments in AI happening in the next 12 months. The full report is a must-read for anyone involved in the field.
Quite unsurprisingly, the report confirms what we've known all along: GPT-4 knows no rivals, and proprietary generative AI has defied the emerging trend noted in the 2022 report, where the authors believed the field was on its way to decentralization because closed models were being implemented and improved upon by the open source community much faster than expected. Fortunately, the open-source community thrives, and the releases keep coming, with the efforts now focused on achieving performance comparable to GPT-4 but using smaller models and higher-quality data sets.
The open source community's mission feels especially urgent if one considers that the news has broken that GPT-4 tests better than every other model but also better than some humans. For instance, while GPT-3.5 scored a mere 10% in the Uniform Bar Exam, the standardized test prospective lawyers in the US have to approve before becoming licensed for legal practice, GPT-4 scores 90%. This would mean that only about 10% of the human test-takers can fare better than GPT-4. (It is fascinating to find out that among the few tests where GPT-4 ranks below 60%, one can find the AMC 1o, AP English Literature and English Language, and GRE Writing.) Even so, GPT-4 still hallucinates, although less than the best-performing predecessor.
GPT-4's success confirms yet another suspicion that has been making the rounds for a while now: reinforcement learning from human feedback (RLHF) is the key to developing state-of-the-art LLMs, especially those with chat applications. The downside, and perhaps one contributing to the divide between closed and open-source AI development, is that RLHF is expensive, difficult to scale, and affected by bias. The superiority of RLHF was also reinforced when research uncovered that not all models benefit from imitation data, thus limiting the pool of alternatives to RLHF when trying to achieve or surpass closed model performance. This will be an uphill battle, especially considering how the 2023 report announces the death of the technical report. As the field gets more competitive and security concerns increase, the research behind proprietary models becomes opaque, an exception being Meta AI's LlaMA family of models.
The turn to smaller models is also partially driven by the increasing computing power demand. The ever-growing computing requirements of AI have sent NVIDIA into the $1T market cap club, consolidating its status as the most popular manufacturer of AI chips. And even when the US has placed restrictions on chip exports, several manufacturers have found a way to market hardware that flies under the radar, thus unlocking the Chinese market. Meanwhile, players in the field, big and small, are boasting their GPU infrastructure as a selling point. After seeing over $18 billion raised in VC funds by AI-focused startups, the team behind the report predicts that the next 12 months will bring GPU debt funds launched by financial institutions as an alternative to VC equity dollars for compute funding.
Security concerns have finally entered the mainstream. The existential risk is as present as ever, and discussion has intensified in the past 12 months. Governments are getting involved and trying to take a stand on the risk of generative AI. Still, there is a long way to go before global governance, with the current landscape being peppered with fragmented approaches to AI security and risk mitigation. Furthermore, the open vs closed model competition has given rise to a dilemma: open-source models are more susceptible to misuse. On the other hand, proprietary models may be more secure but less transparent.
On top of this, there are inherent security risks within RLHF, and models are rapidly exceeding expectations on output and capabilities, which makes them progressively harder to monitor. Institutions are experimenting with model-assisted supervision and self-improving models. The success of these measures seems to be tied to another obstacle we've heard about constantly: Benchmark-based evaluation is not consistent enough, as metrics are tied to implementation. Regardless, self-improving agents seem promising enough to figure in one of the report's predictions for the following 12 months.
There is a lot more to cover, especially since the report also attempts to bring other less popular AI applications into the spotlight, including but not limited to self-driving vehicles, drug discovery, and medical and pharmaceutical research, in addition to in-depth politics and industry discussion.