YOLO-World: Real-Time Open-Vocabulary Object Detection
YOLO-World boosts YOLO with open-vocabulary detection via vision-language modeling, pre-training on large datasets. Efficiently detects objects zero-shot, outperforming state-of-the-art in accuracy and speed.
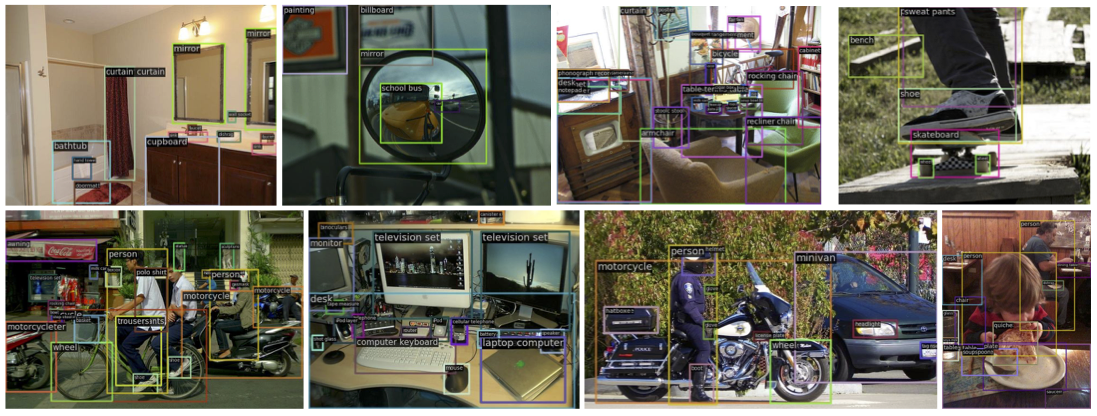
Tianheng Cheng2,3,* , Lin Song1,*,📧 , Yixiao Ge1,2,⭐ , Wenyu Liu3 , Xinggang Wang3,📧 , Ying Shan1,2
1 Tencent AI Lab, 2 ARC Lab, Tencent PCG, 3 Huazhong University of Science and Technology
Abstract
The You Only Look Once (YOLO) series of detectors have established themselves as efficient and practical tools. However, their reliance on predefined and trained object categories limits their applicability in open scenarios. Addressing this limitation, we introduce YOLO-World, an innovative approach that enhances YOLO with open-vocabulary detection capabilities through vision-language modeling and pre-training on large-scale datasets. Specifically, we propose a new Re-parameterizable Vision-Language Path Aggregation Network (RepVL-PAN) and region-text contrastive loss to facilitate the interaction between visual and linguistic information. Our method excels in detecting a wide range of objects in a zero-shot manner with high efficiency. On the challenging LVIS dataset, YOLO-World achieves 35.4 AP with 52.0 FPS on V100, which outperforms many state-of-the-art methods in terms of both accuracy and speed. Furthermore, the fine-tuned YOLO-World achieves remarkable performance on several downstream tasks, including object detection and open-vocabulary instance segmentation.
Framework
- The YOLO-World builds the YOLO detector with the frozen CLIP-based text encoder for extracting text embeddings from the input texts, e.g., object categories or noun phrases.
- The YOLO-World contains an Re-parameterizable Vision-Language Path Aggregation Network (RepVL-PAN) to facilitate the interaction between multi-scale image features and text embeddings. The RepVL-PAN can re-parameterize the user's offline vocabularies into the model parameters for fast inference and deployment.
- The YOLO-World is pre-trained on large-scale region-text datasets with the region-text contrastive loss to learn the region-level alignment between vision and language. For normal image-text datasets, e.g., CC3M, we adopt an automatic labeling approach to generate pseudo region-text pairs.
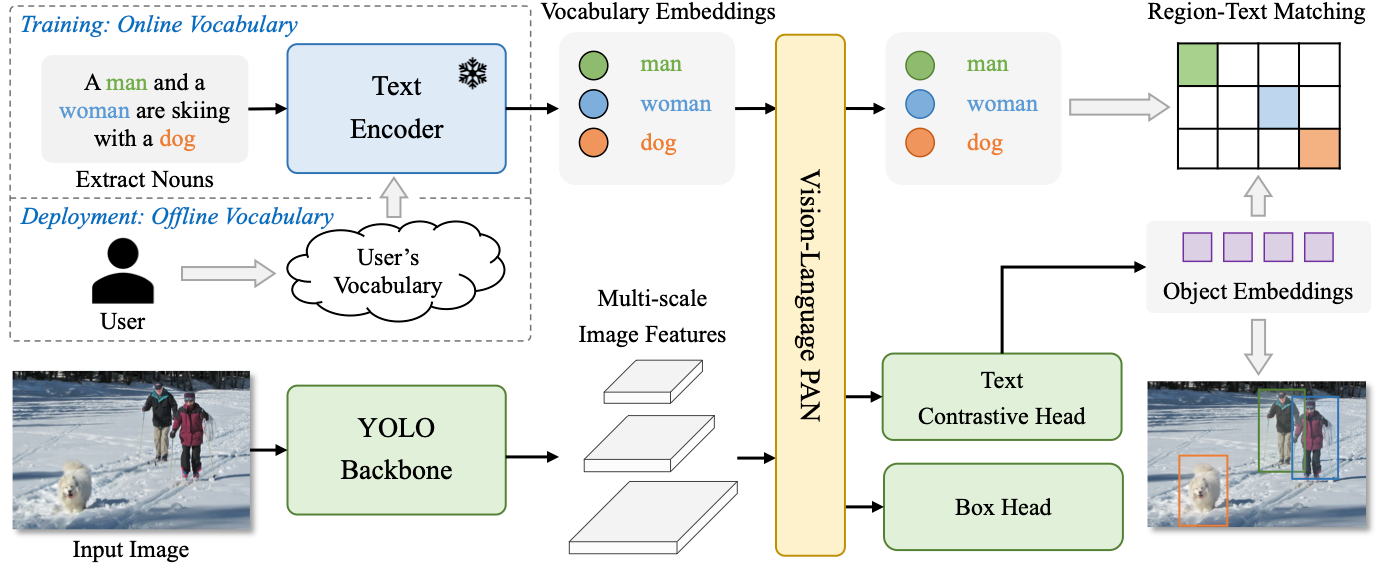
Please check more details in our technical report.