YOLOv11, the latest in the Ultralytics YOLO series, is remarkably flexible and versatile
YOLOv11 is an advanced iteration of the YOLO object detection series that offers enhanced feature extraction capabilities, improved computational efficiency, and various specialized models for different computer vision tasks while maintaining flexibility across deployment environments.
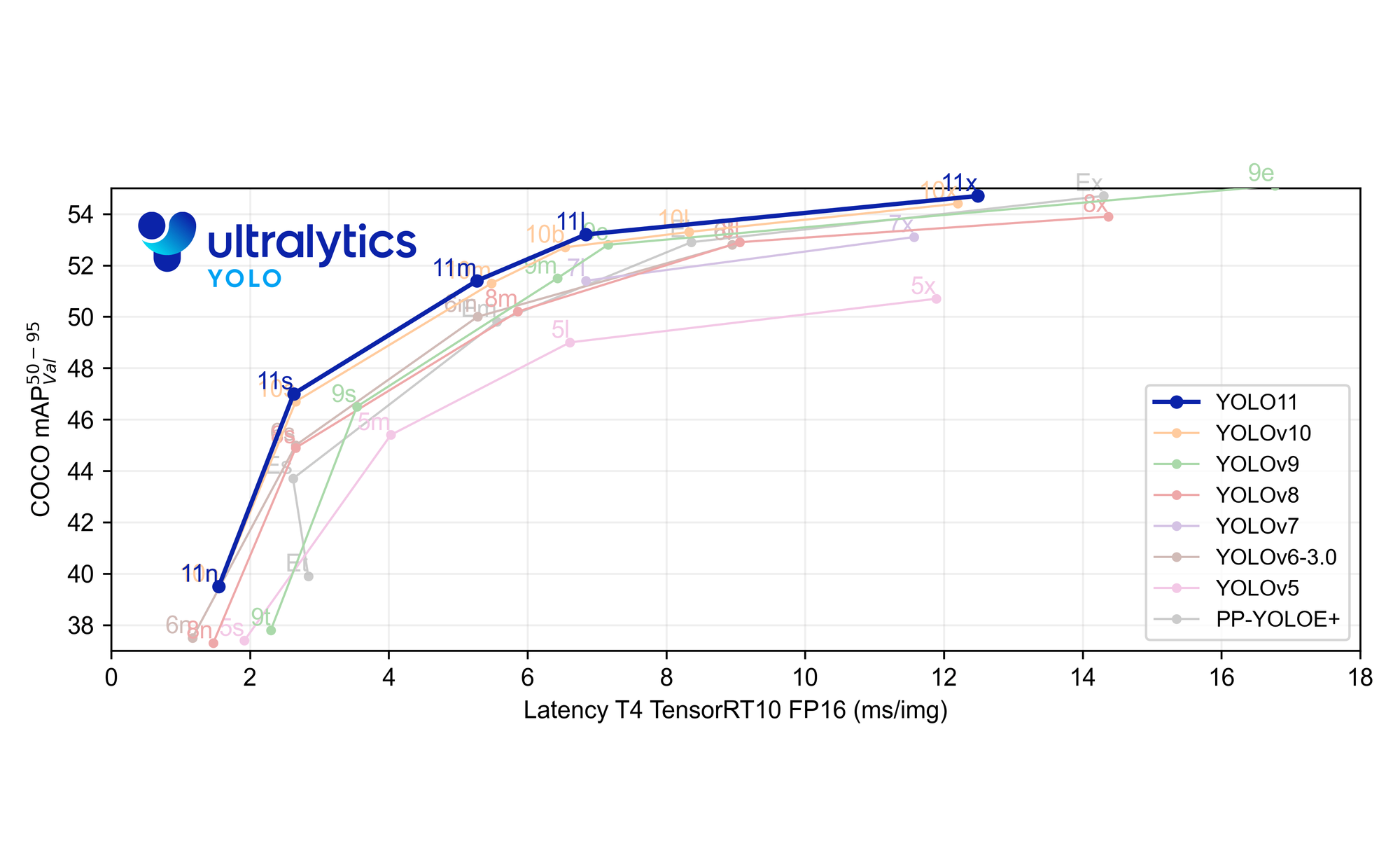
YOLOv11 builds on the success of its predecessors and thanks to significant advances in its architecture and training methods, this iteration of the popular real-time object detector series is set to become an attractive and versatile choice for several computer vision tasks, including object detection, instance segmentation, and image classification. YOLOv11's most noteworthy improvement over previous versions is its enhanced feature extraction capabilities. As is well known, feature extraction is an essential ingredient of highly accurate object detection. YOLOv11's improved architecture enhances feature extraction, improving object detection accuracy and unlocking a strong performance in complex computer vision tasks.
In addition to its enhanced feature extraction capabilities, YOLOv11's advances in its architecture and optimized training pipelines ensure faster processing speeds without loss of accuracy. In particular, YOLOv11m demonstrates its computational efficiency when compared with YOLOv8m by achieving a greater mean Average Precision (mAP) on the COCO dataset using 22% fewer parameters.
YOLOv11 inherits the flexibility and versatility of its predecessors: YOLOv11 follows the same strategy of introducing a range of models specializing in different computer vision tasks: YOLO11 for detection, YOLO11-seg for instance segmentation, YOLO11-pose for pose estimation, YOLO11-obb for oriented detection and YOLO11-cls for classification. Notably, these models can operate in Inference, Validation, Training, and Export modes, further supporting YOLOv11's applicability in varied and complex computer vision tasks.
In parallel, the YOLOv11 model range also incorporates the size variations that make YOLO11 deployable across several environments, from edge devices, and cloud platforms, to systems supporting NVIDIA GPUs. Each task-specialized model has variants across all the available sizes. YOLOv11n (n for nano) is the smallest version of the model range, appropriate for extreme resource constraints, such as the ones found in edge devices. YOLOv11s (small) delivers exceptional speed while maintaining accuracy, YOLOv11m is a medium-sized model for general-purpose applications, and the YOLOv11l and YOLOv11x variants deliver the highest accuracy at the expense of higher computational resource consumption.
Performance metrics, usage examples, and links to all the relevant documentation for in-depth coverage of each of the YOLOv11 variants can be found here.