Large-scale text-driven fusion diffusion models have attracted a lot of attention because of their remarkable ability to generate a wide variety of images that follow given text cues. Based on these fusion models, it has become natural to create text-driven image editing capabilities. But because it is an inherent property of editing techniques to retain some of the content of the original image, whereas in text-based models, even a small change to a textual cue often leads to a completely different result, such editing is a challenge for these generative models.
Google Research, together with researchers from Tel Aviv University, have created a method that mitigates this problem by requiring users to provide a spatial mask to localize the edit, hence ignoring the original structure and content within the masked area. In this work, they use an intuitive prompt-to-prompt editing scheme, where editing is controlled only by the text.
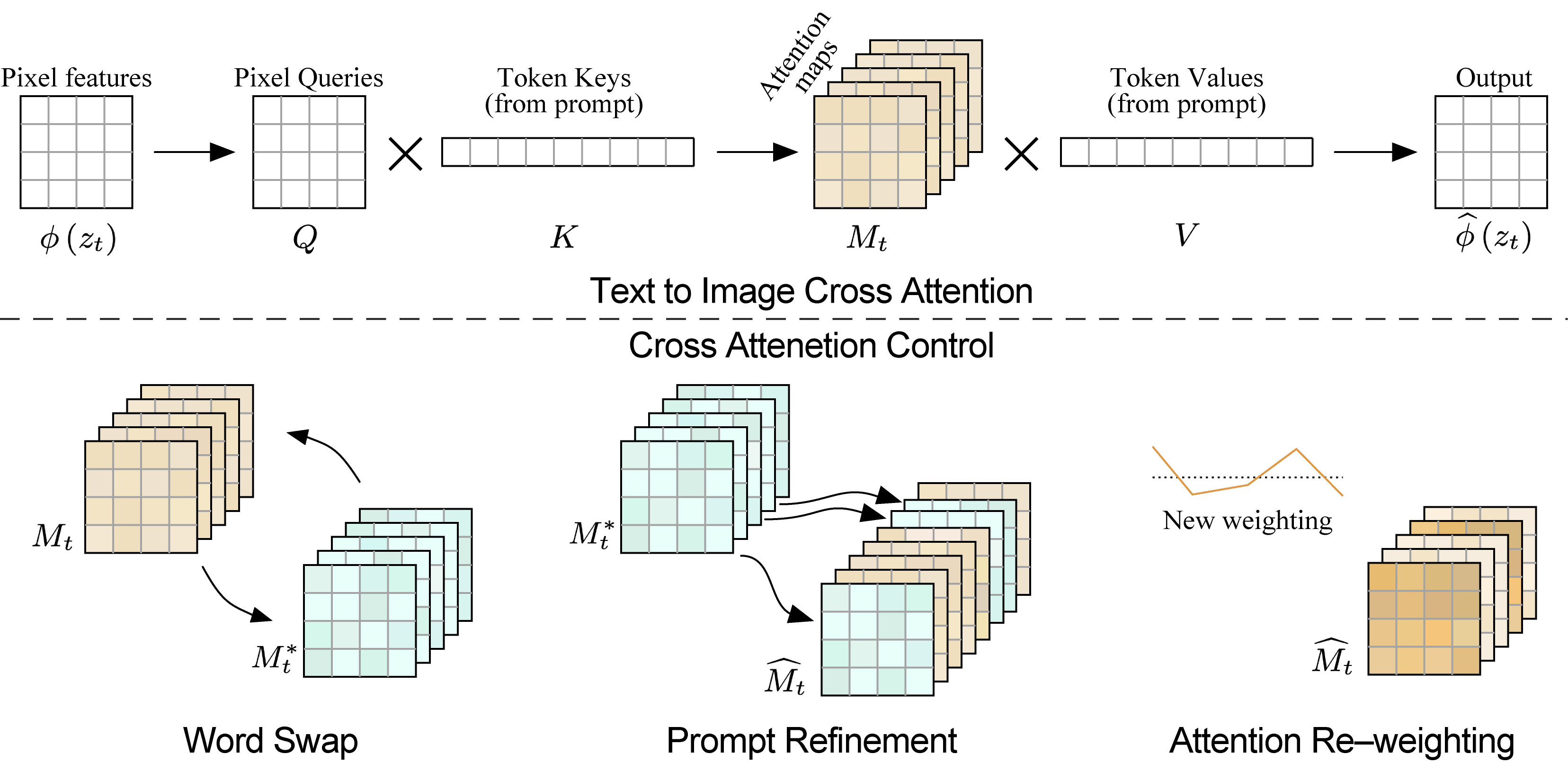
Thus, first there is a detailed analysis of the text-conditioned model and the observation that layers of cross-attention are key to controlling the relationship between the spatial location of the image and each word in the cue. Given this observation, the method proposes to control the attention maps of the edited image by introducing the attention maps of the original image into the diffusion process.
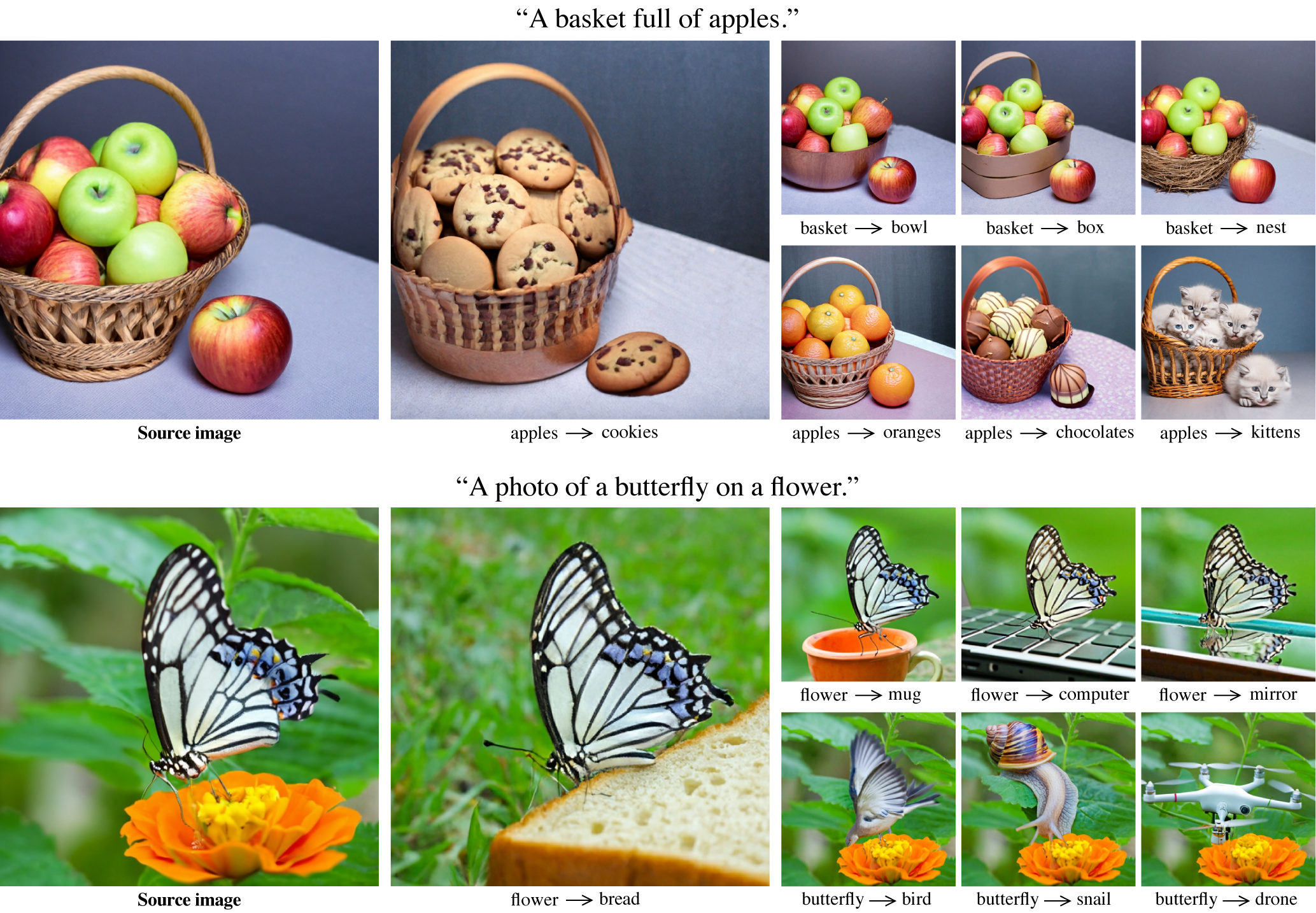
This approach allows controlling the fusion process by editing only the text cue, which opens the way to a huge number of applications for caption editing, such as localized editing by word replacement, global editing by adding refinement, and even controlling the degree of word reflection in the image.
- Project - https://prompt-to-prompt.github.io
- Paper - https://prompt-to-prompt.github.io/ptp_files/Prompt-to-Prompt_preprint.pdf
- Code - https://github.com/google/prompt-to-prompt/
We hope you haven't forgotten the last pager about LION - latent point diffusion models for generating 3D shapes. This is a DDM for 3D shape generation that focuses on training a 3D generative model directly on geometric data without image-based learning.
Comments