LION: Latent point diffusion models for generating 3D shapes
Diffusion denoising models (DDM) have shown promising results in the synthesis of 3D point clouds. For 3D DDM to get better, it requires high quality generation, flexibility for manipulation and applications such as conditional synthesis and shape interpolation, and the ability to output smooth surfaces or meshes. At the NeurIPS
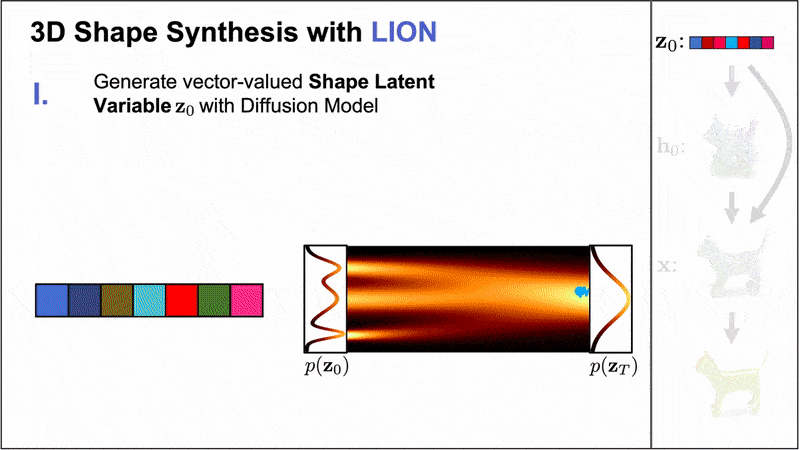
Diffusion denoising models (DDM) have shown promising results in the synthesis of 3D point clouds. For 3D DDM to get better, it requires high quality generation, flexibility for manipulation and applications such as conditional synthesis and shape interpolation, and the ability to output smooth surfaces or meshes.
At the NeurIPS 2022 conference, a novelty in the AI world is presented, the Latent Point Diffusion Model (LION). This is a 3D shape generation DDM that focuses on training a 3D generative model directly on geometric data without image-based learning. Like previous 3D DDMs in the field, LION works with point clouds, but it is built like a VAE with a DDM in latent space.
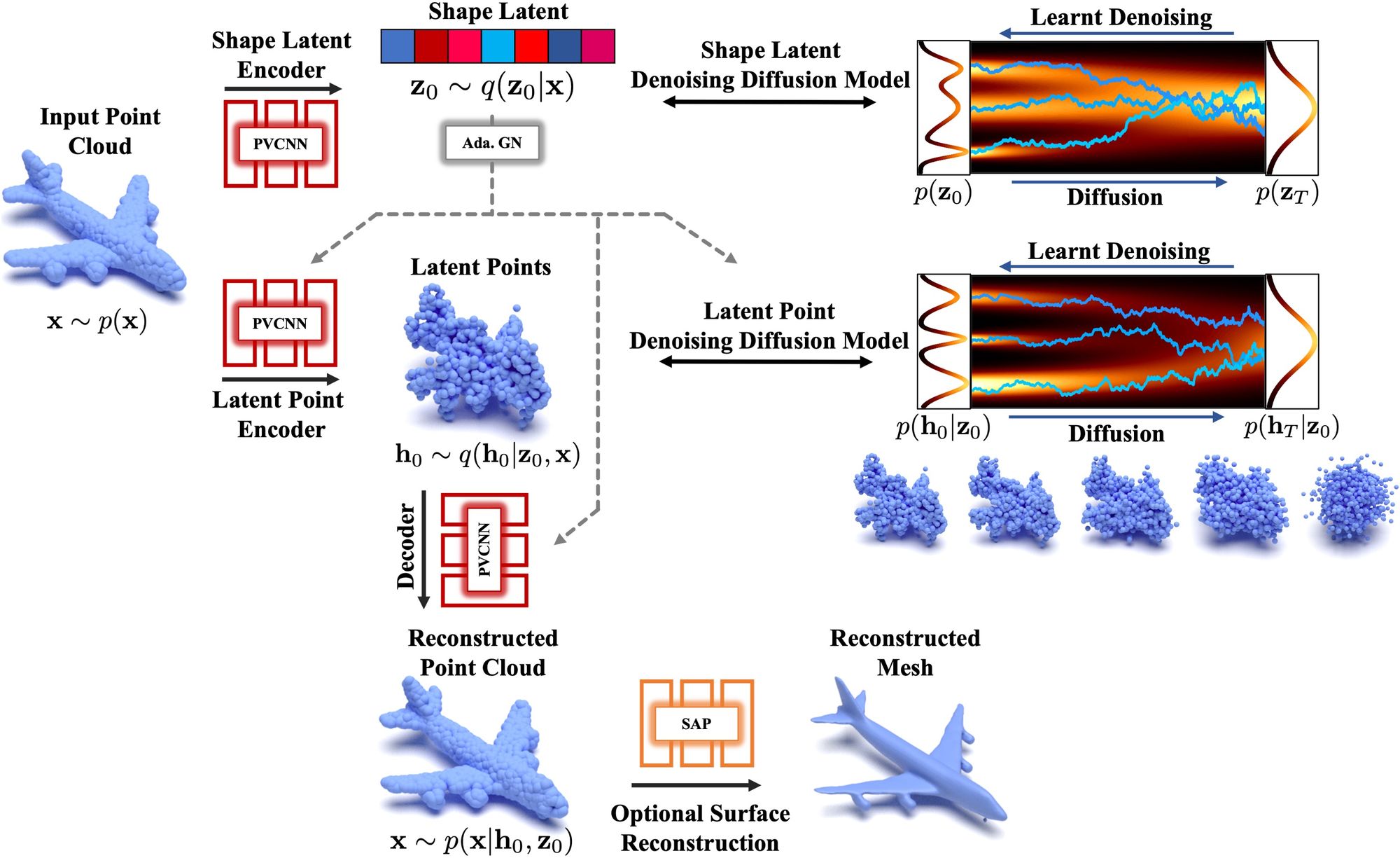
LION includes a hierarchical latent space with a vector-valued global shape latent space and another latent space with a point structure. Latent representations are predicted using point cloud processing coders, and two latent DDMs are trained in these latent spaces. Synthesis in LION occurs by extracting new latent samples from the hierarchical latent DDMs and decoding back into the original point cloud space.
The main advantages of LION: expressiveness - when due to the mapping of point clouds into regularized latent spaces, the DDM in the latent space is effectively trained in a smooth distribution; different types of output - LION extension with Shape As Points (SAP) geometry reconstruction also allows to output smooth meshes; flexibility - because LION is designed as VAE, it can be easily adapted to different tasks without re-training the latent DDM.
The main goal of the developers was to create a high-performance generative model of 3D shapes. LION can be used for many interesting applications: shape interpolation, fast sampling with DDIM, multimodal generation, voxel-conditioned synthesis, single view reconstruction, texture synthesis from samples based on text.
- Project - https://nv-tlabs.github.io/LION/
- Paper - https://arxiv.org/abs/2210.06978
- Code - https://github.com/nv-tlabs/LION
In a previous paper, we introduced Omni3D, a large benchmark and model for detecting 3D objects in the wild, which uses and merges existing datasets, resulting in 234,000 images annotated with more than 3 million instances and 97 categories.