The distinction between 'open' and 'closed' (or proprietary) technologies has become entrenched in the current ecosystem of large language models and generative AI technologies. Moreover, it is unquestionably clear what it means for a technology to be closed; that details about its inner workings are inaccessible to an audience wider than the owners of said technology. In contrast, what someone may mean by calling a technology or model 'open' is becoming increasingly obscure. This is because models may be publicly released under the guise of being open-source, albeit under ambiguous licenses; or just enough of their components may be released under a true open-source license so everyone may leverage them for personal and commercial uses free of charge, but further details and key components like training datasets remain closed, so the wider audience cannot gain any insights into how the model works, nor can there be any reproducibility of the reported benchmark scores.
The more suspicious of these practices, sometimes known as 'open-washing', are enabled mainly because there is no agreed-upon standard to refer to when evaluating the actual openness of a model. The Linux Foundation AI & Data is releasing the Model Openness Framework (MOF) to mediate this issue. The MOF is a comprehensive evaluation framework for the completeness and openness of machine learning models. The MOF identifies 16 critical components and evaluates whether each was released using an appropriate open license according to the artifact type. Then, it defines three classes according to the components released under true open-source licenses.
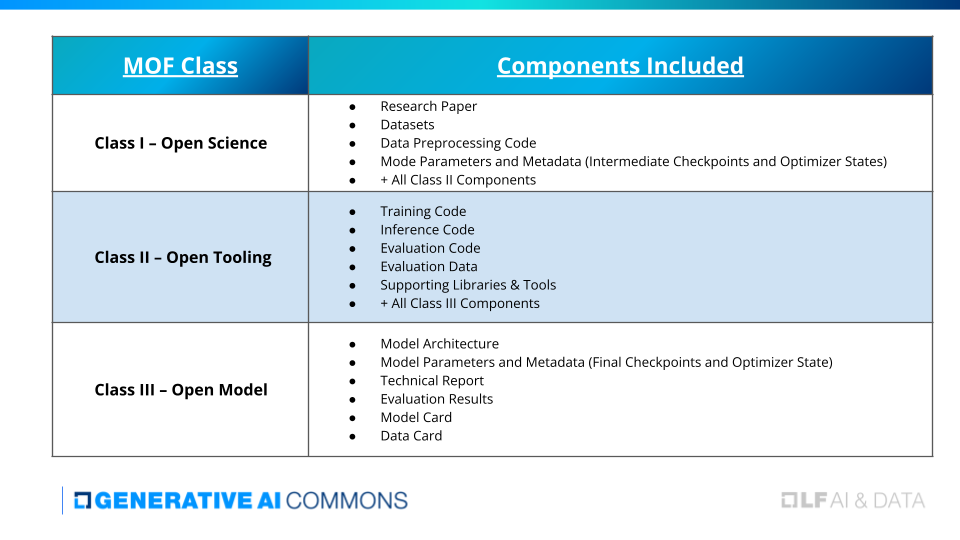
As can be seen from the table, Class III - Open Model lays the minimum standard, Class II - Open Tooling enables some degree of reproducibility, and Class I - Open Science entails the release of the 16 critical components under open-source licensing. As a result, Class I models are fully transparent, enabling full reproducibility, collaboration, and building on previous landmarks. Generally, the MOF aims to bring the teachings and successes of open science and open-source software movements to generative AI. It also builds upon the existing innovative open-source AI initiatives such as Eleuther AI's or those from the Allen Institute for AI.
To contribute to the advancement of the MOF and related initiatives, anyone interested can join the Generative AI Commons, an open and neutral forum for advancing open-source AI and open science. The Generative AI Commons is working on the upcoming Model Openness Tool (https://isitopen.ai/) and a badging system to certify the openness level of GitHub-hosted projects.
Comments