ULNeF: Untangled Layered Neural Fields for Mix-and-Match Virtual Try-On
Recent advances in neural models have shown excellent results for virtual fitting tasks (VTO), where a 3D representation of a garment is deformed to fit the target body shape. However, existing solutions are limited to a single layer of clothing and cannot solve the combinatorial complexity of mixing different types
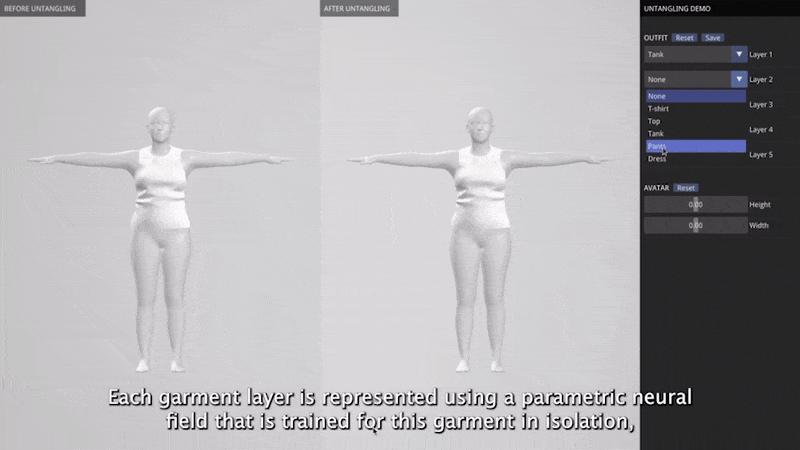
Recent advances in neural models have shown excellent results for virtual fitting tasks (VTO), where a 3D representation of a garment is deformed to fit the target body shape. However, existing solutions are limited to a single layer of clothing and cannot solve the combinatorial complexity of mixing different types of clothing.
To address this limitation, scientists present non-overlapping layered neural fields, ULNeFs, which solve the multi object interaction problem using implicit object representations. They represent multiple possibly colliding objects (e.g., multiple items of clothing) using a layered version of the neural fields and develop an algorithm that unravels these layered neural fields to represent objects without collisions.
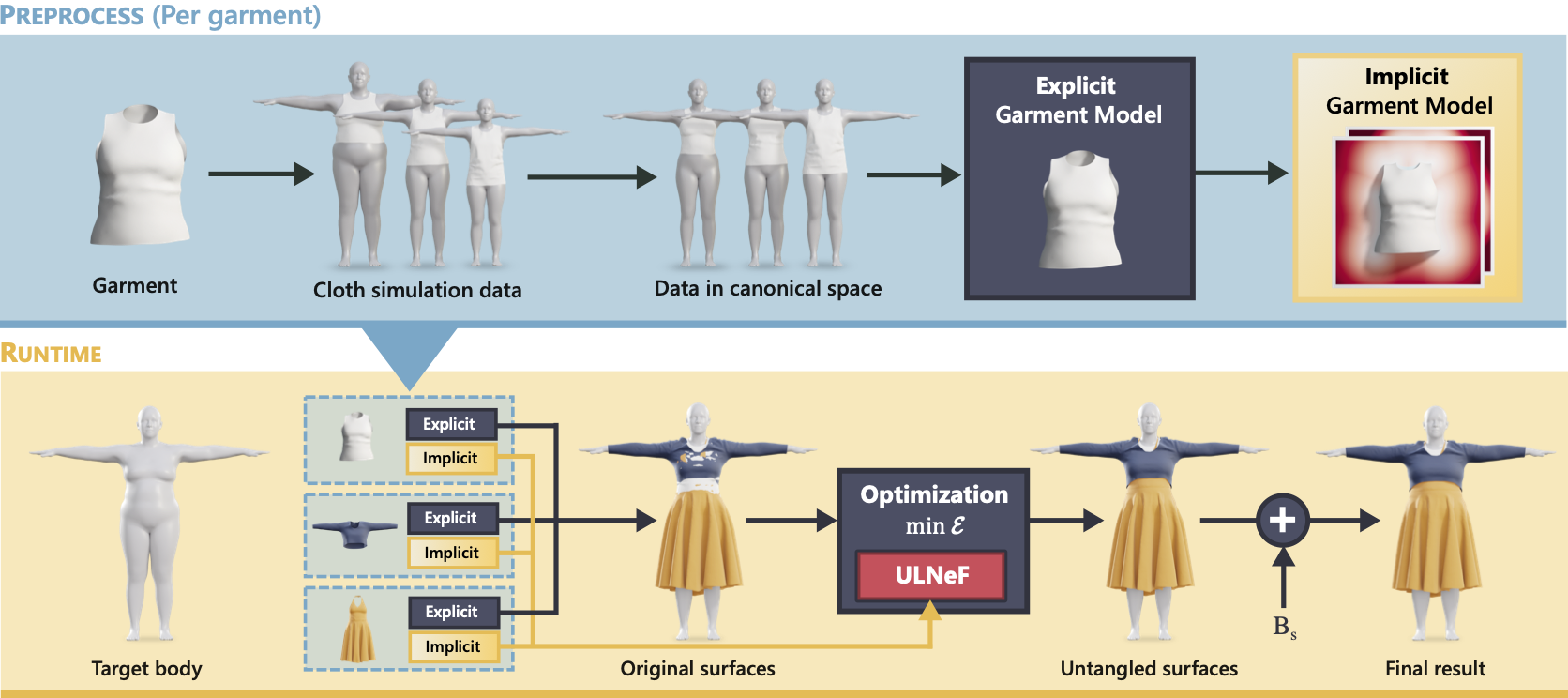
What happens is that they first learn two fields: a landmark distance field f(x), which represents the surface of a garment and gives the notion of "inside-outside," and a covariant field h(x), which models the volume near holes through which other garment objects can pass without creating entangled configurations. Using these fields, it is possible to determine whether point x is in a tangled configuration. And thanks to the implicit surface representation, untangling can be formulated as a local operation on the values of the fields at points x.
This state-of-the-art neural projection operator is trained only once for any arbitrary combination of N surfaces, and after training it naturally generalizes to any garment or implicit surface.ULNeFs can produce order-dependent results, depending on how the layers are sorted.
- Project - http://mslab.es/projects/ULNeF/
- Paper - http://mslab.es/projects/ULNeF/contents/santesteban_NeurIPS2022.pdf
And the new generation of AI's generative content creation tool, AI eDiff-I, offers unprecedented text-to-image fusion, instant style transfer, and intuitive word-drawing capabilities. This diffusion model of image fusion from text is based on T5 text inserts, CLIP image inserts, and CLIP text inserts. This approach allows you to generate photorealistic images that match any textual query.