EvoDiff, Microsoft’s protein-generating AI, is now open source
Microsoft’s protein-generating diffusion model, EvoDiff, is now open source. The groundbreaking model generates protein sequences rather than 3D structures, allowing its output to be more general, flexible, and realistic than that of other similar models.
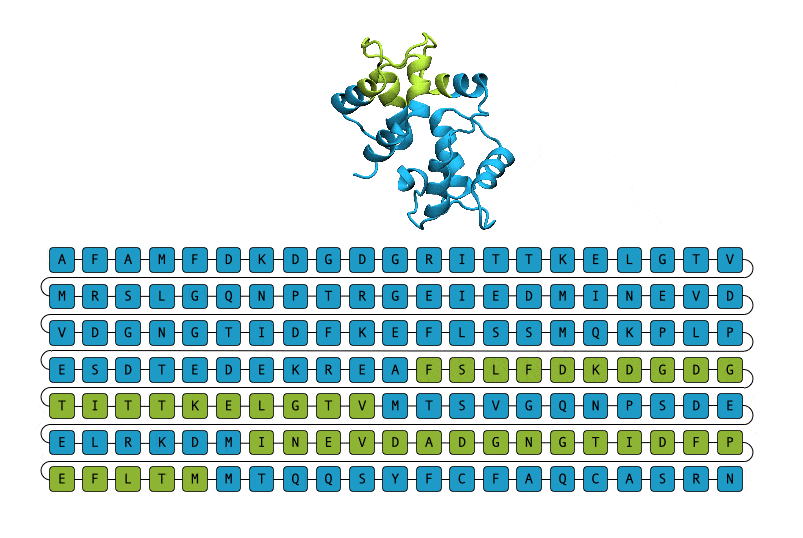
Proteins are the biomolecules responsible for a variety of processes not just in our bodies, but in nature. We are familiar with proteins that play a role in essential bodily functions, such as hemoglobin carrying oxygen through the bloodstream or insulin helping in the regulation of blood sugar levels. Proteins are also the building blocks of hair, nails, and muscular tissue. We now know that some proteins have an important role in the progression of disease. And outside of the human body, proteins are involved in a range of chemical reactions so wide, some of them have become part of our daily lives. Enzymes are a family of proteins best known for their ability to break down molecules and their power has already been harnessed in the production of compost and detergents.
Given their importance and ubiquity, it is unsurprising that researchers would want to turn to protein generation techniques as a means to discover new proteins to advance the generation of therapeutics and catalysts, and even gain insights into the process and treatment of some diseases. Unfortunately, the process of protein generation in a laboratory setting has proven to be a resource-intensive process. Proteins are composed of a chain of amino acids, best thought about as a sequence of smaller building blocks. This chain often folds into a 3D structure to be able to carry out its assigned function.
Thus, research into protein generation frequently begins by coming up with a structure that could plausibly play a role in the studied process and then generate candidate proteins that could fold into the desired structure. This is a slow and expensive process, partly because there are more sequences than structures, and more than one sequence can fold into a given structure. Furthermore, these methods are largely ineffective when it comes to generating disordered proteins, which do not need to fold into a given 3D structure to fulfill their function. Disordered proteins are especially important since they are known to be involved in the process of disease.
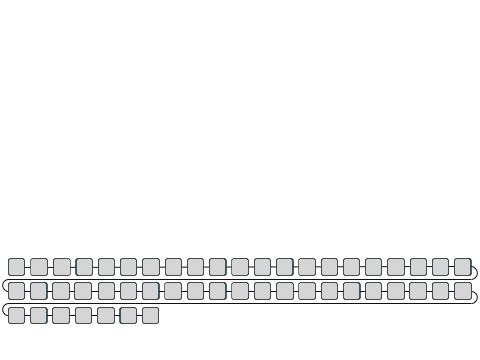
Enter EvoDiff, Microsoft’s diffusion protein generation model. Unlike other protein-generation methods, EvoDiff generates protein sequences directly, which makes it ideal for the generation of realistic disordered proteins. Moreover, EvoDiff is also innovative because it is the first model trained on evolutionary data. Diffusion models for protein generation are not new, but according to Dr. Kevin K. Yang, one of the researchers behind EvoDiff, other models are usually trained on small sets of related protein data, severely limiting the kinds of proteins the model can generate. The research team hopes that evolutionary data will lead to “a model that is hopefully universal or as close to universal as we can get for protein sequence space.”
Another of EvoDiff’s groundbreaking features is the capability to fine-tune the output by adding context to the process. According to Dr. Ava Amini, the team asked themselves, “if we give some context to the model, a little bit of information, can we guide the generation to fulfill particular properties that we want to see in that protein?” It turned out they could give the model information about another protein they wanted the generated protein to bind to, and the model learned to restrict its output accordingly, producing only sequences that satisfied the additional requirement.
EvoDiff definitely offers reason to be excited about the future, but the research team behind it is well aware that the model still has a long way to go. Their paper has not undergone peer-review, being available only as a pre-print. Furthermore, the viability of the generated sequences has not been yet tested even in a laboratory setting. The team is also looking to scale the model up both in the amount of training parameters and the types of context that can be offered. The team reportedly plans “to condition EvoDiff on text, chemical information or other ways to specify the desired function.”