The open-source LLM ecosystem flourished in 2023
ChatGPT's coming to existence will go down into the history books as the catalyst for the LLM race that dominated 2023. Fortunately, ChatGPT also ushered in the creation of the open-source ecosystem, which has given us powerful yet compact LLMs and shows no signs of stopping.
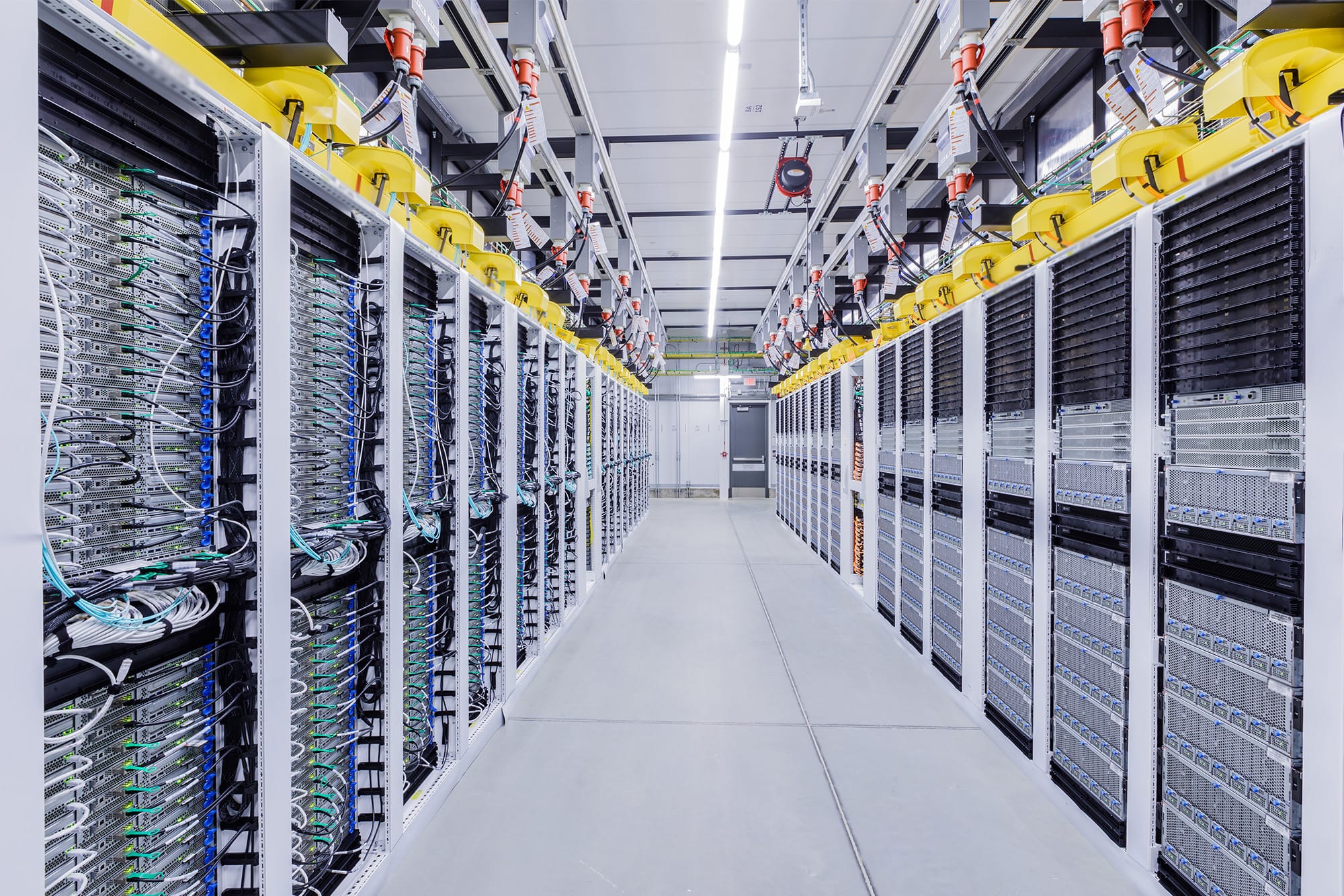
ChatGPT's coming to existence will go down into the history books as the catalyst for the LLM race that dominated almost all of 2023. Not only did the chatbot's availability fundamentally change how people and computers interact, but it also ushered in an era of closed walls, secrecy, and proprietary services as AI companies and tech giants battled it out to dominate the AI-powered applications market. This translated into companies selling API access to their models, revealing as little as possible about underlying model weights, training sets, and methodologies.
Even today, there is lingering speculation about GPT-4's actual architecture and number of parameters: according to the rumors, GPT-4 has a mixture of experts architecture, which means it is built out of either eight 220B-parameter models or 16 models with 110B parameters each. In any case, the count would put GPT-4's total parameters at over 1 trillion. The speculation surrounding the specifics of proprietary models led to another dominating belief in the LLM wars: bigger is always better. Indeed, the competition was driven by the idea that the only possible way to reach, or even surpass, GPT's performance was by training models requiring colossal datasets and ever-increasing amounts of computing power.
The thought that massive was undoubtedly better became so pervasive that even open-source models comparable to GPT-3, such as BLOOM and OPT, even if publicly accessible, require substantial computing resources nearly impossible to come by. Fortunately, after Meta introduced the initial LLaMA family to the open-source ecosystem in February 2023, everyone realized that smaller models trained on bigger datasets could achieve competitive performance without needing an unusually substantial number of parameters or the computing power hunger that still characterizes closed proprietary models. Where GPT-3 is believed to be trained on around 300B data tokens, the LLaMA models report a training dataset of approximately 1.4T tokens, nearly five times as many.
Meta's training strategy and its models' availability and capacity to run on a modest number of dedicated GPUs (sometimes, even on a single one) paid off. Researchers were able to build on Meta's findings, and thus, the emergence of powerful yet compact LLMs such as MosaicLM's MPT or TIIUAE's Falcon began. These models were soon joined by Meta's LLaMA 2, which fostered the creation of thousands of derivatives. Then, just in time for the holidays, Mistral AI recently followed up its September release of Mistral 7B with Mixtral 8x7B, a high-quality sparse mixture of experts (SMoE) model that matches or outperforms LLaMA 2 70B and GPT-3.5 on several standard benchmarks. Despite the recency of Mixtral's launch, the search term "Mixtral" already returns over 400 results at Hugging Face.
The influence of the open-source community proved to be so strong that even Amazon and Microsoft, known backers of the industry-leading titans Anthropic and OpenAI, respectively, demonstrated their receptiveness by working on the integration of open models in their services: Azure AI Studio platform can be used to build AI applications based on open-source models, while Amazon Bedrock can host both proprietary and open models. Additionally, Microsoft even released its open-source models Orca and Phi-2.
Open-source models also found an unexpected edge given the proprietary services' reliance on external APIs. Although this method of granting access to services has several benefits, it poses a significant privacy-related risk: even if data leaks may be unlikely or even preventable, they are never impossible. The capability of deploying an open-source model on-premises eliminates the need to upload sensitive data to the cloud, thus giving a safer option to those companies prioritizing data safety and privacy. For instance, cybersecurity startup Nexusflow harnessed the power of the LLaMA models to build NexusRaven-13B, the custom model powering their cybersecurity Copilot.
As governance and compliance concerns continue to heighten and the European Union hastens to become the world's AI compliance enforcement agency, it seems as if the open-source ecosystem will keep gaining traction in the coming year. Moreover, we are sure that privacy and security are among the many possibilities that remain open for the open-source community to innovate and flourish. If 2023 was any indication, the community is also primed to continue its role as a powerful driver of scientific progress. Here's to hoping that 2024 will bring even more exciting developments in AI that are accessible to everyone.