MIT looks to improve the quality of life for cerebral palsy patients using a pose-mapping technique
A team of MIT engineers looks to make clinical evaluation of cerebral palsy patients easier and remotely accessible. They’ve developed a method that analyses videos of patients and assigns them a score based on the presence of movement patterns specific to the development of the disorder.
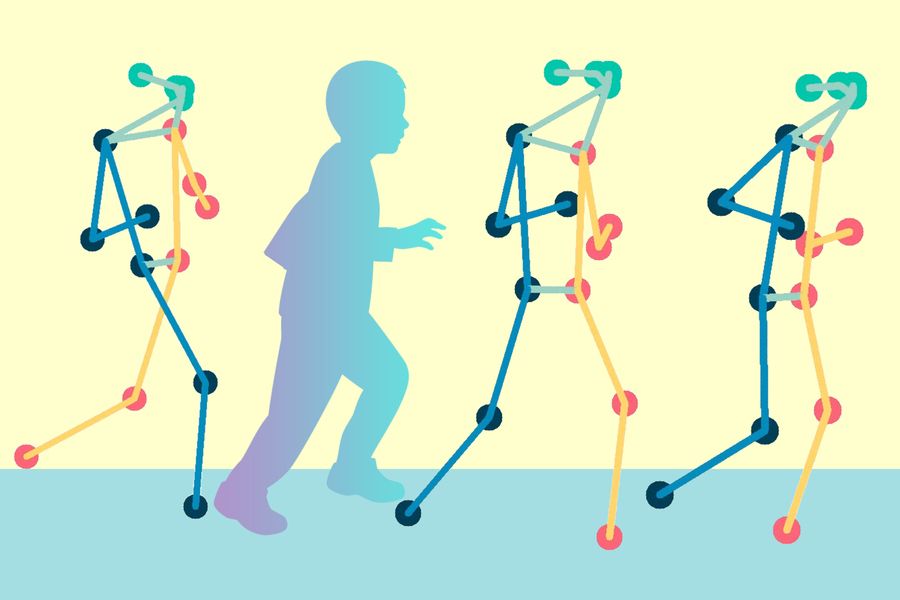
Patients with motor disorders such as cerebral palsy often have to undergo frequent in-person evaluations so doctors can monitor the development of the disorder. For parents of children with cerebral palsy, regular doctors’ appointments can quickly turn into a costly, time-consuming, and stressful routine. A group of MIT engineers is looking to change this by developing a remote diagnosing technique that is comparable to an in-person medical screening.
In a nutshell, the diagnosing technique combines computer vision and machine learning to offer a real-time analysis of patients’ videos. The method then calculates a clinical score based on whether it identifies certain patterns of poses in the video frames. To test their method, researchers took videos of over a thousand children with cerebral palsy. The method’s scores had an accuracy of over 70% when compared to the diagnosis of a medical professional done in a clinical setting.
The pose-mapping technique can be run on a variety of smartphones and tablets. The engineering team’s ultimate goal is that patients could receive everyday monitoring by simply setting up their phone or tablet to take video at home. They could then run the videos through a program for analysis and scoring. The same videos and progress scores could then be forwarded to the doctor’s office for review and confirmation. The team also expects to be able to extend their method to the evaluation of other motor disorders.
The method begins with a pose estimating algorithm. These algorithms take videos of human movement as input and translate them to a series of skeleton poses, which are visualized as a structure of lines and dots for scientists to further analyze. The engineering team took a skeleton pose dataset made available by Stanford University’s Neuromuscular Biomechanics Laboratory comprising the videos of over a thousand children with cerebral palsy. In each video, the children perform a series of exercises supervised in a clinical setting. The videos are also tagged with the Gross Motor Function Classification System (GMFCS) score assigned to them in the corresponding in-person evaluation. The Stanford researchers ran each video through a pose estimating algorithm to create the dataset that became the starting point for the method.
The second step in the method consists of deciphering the movement patterns specific to the different stages of cerebral palsy. To do this, the MIT research team took a model pre-trained on video data of healthy adults performing daily activities and fine-tuned it on the skeleton data from the Stanford data set. The use of a pre-trained model led to more accurate scores than using a model trained exclusively on cerebral palsy data. Principal researcher Zhao explains, “Because the network is trained on a very large dataset of more general movements, it has some ideas about how to extract features from a sequence of human poses.”
The team is confident that their research will be life-changing for patients of a variety of motor disorders, including Parkinson’s. They are already planning to condense their findings into a mobile app that would enable patients to be evaluated in the comfort of their own homes. The availability and ease of monitoring will increase patients’ quality of life by reducing the costs and emotional burden associated with frequent in-person evaluations in clinical settings.
MIT’s official announcement can be read here, and the engineering team’s paper is open-access and can be read here.