Silo AI has released Viking, a family of models for all Nordic languages
Silo AI has released Viking, a family of open-source LLMs optimized for Danish, Finnish, Norwegian, Icelandic, and Swedish languages without compromising English language and coding performance.
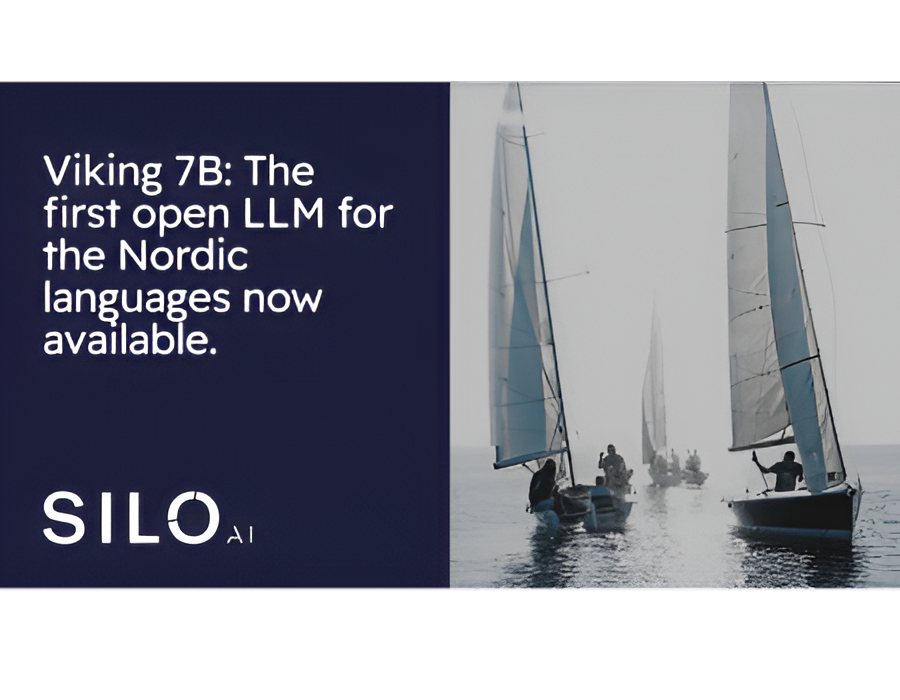
Silo AI's generative AI division, SiloGen, and TurkuNLP of the University of Turku are building on their successful approach to building the Finnish language model Poro to develop Viking, a family of LLMs optimized for Danish, Finnish, Norwegian, Icelandic, and Swedish languages without compromising English language and coding performance. Viking also features an updated architecture and several model sizes. Viking is the latest advancement in Silo AI's plan to empower linguistic diversity across Europe with state-of-the-art models for all official European languages using an approach that focuses on low-resource languages and takes local values and cultures into consideration. The resulting models are meant to build Europe's digital infrastructure and accelerate the adoption of LLM-powered solutions across several industries and use cases throughout the continent.
Evaluations performed after 10 checkpoints, which cover 50% of training and 1000B tokens, show that Viking's multilingual performance is superior to other open-source products in the market, including Falcon, GPT-SW3, Llama, Mistral, MPT, and StarCoder. Viking's outstanding capabilities at understanding and generating Nordic languages and linguistic sequences processing and prediction are evidence of the effectiveness of SiloGen's approach to training multilingual models.
Viking's first release includes five checkpoints, and the model family features an architecture similar to Llama 2, with flash attention, rotary embeddings, and grouped query attention, supporting a 4K-token context window. Current model sizes are 7B, 13B, and 33B, trained on a 1 trillion token dataset representing English, all Nordic languages, and several programming languages. The models were trained using up to 1024 AMD MI250X GPUs on the LUMI supercomputer, and are available for academic and industry research under an Apache 2.0 license.