YOLOv7 by Academia Sinica
The 2016 release of YOLO caused a furor among computer vision professionals. It provided high speed and accuracy for a fundamental problem with applications in autonomous driving, robotics, safety, medical image analysis, and other fields. Then, it also provided multiscale predictions, a better baseline classifier, and other tricks to improve
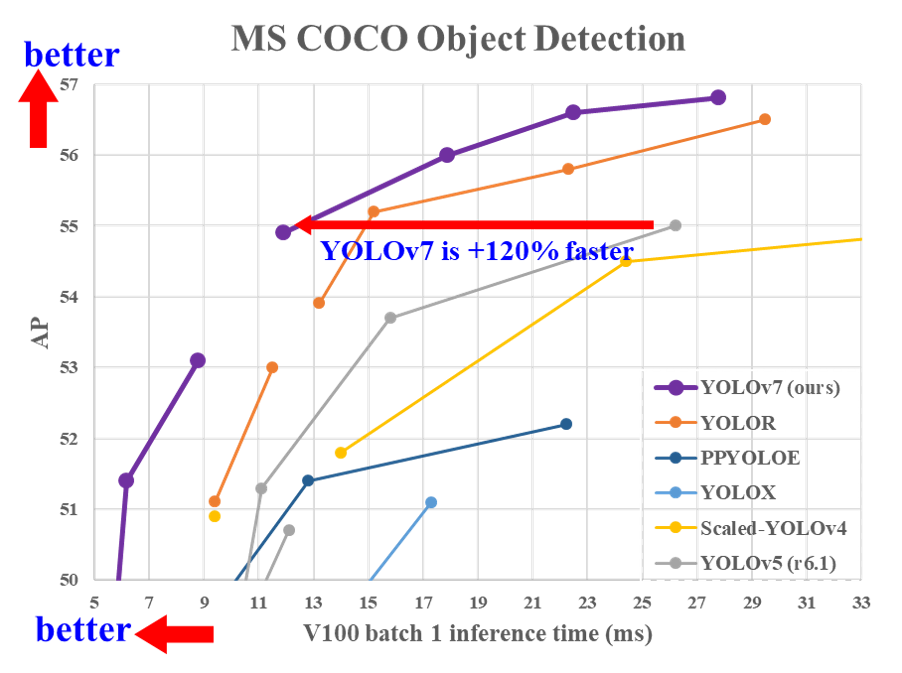
The 2016 release of YOLO caused a furor among computer vision professionals. It provided high speed and accuracy for a fundamental problem with applications in autonomous driving, robotics, safety, medical image analysis, and other fields. Then, it also provided multiscale predictions, a better baseline classifier, and other tricks to improve YOLO learning and performance.
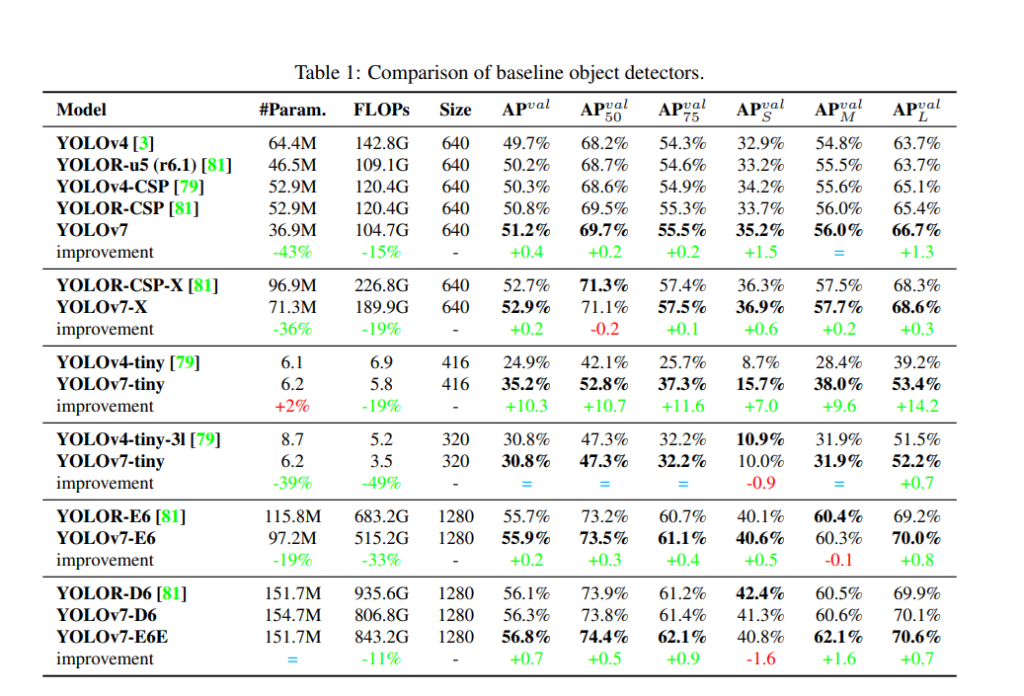
Now, Academia Sinica continues the development of YOLO in its new work YOLOv7, which introduces new methods of "extension" and "combined scaling" — they make efficient use of parameters and computation, and outperform all known real-time object detectors in speed and accuracy.
Thus, the proposed combined scaling method improves the real-time object detection accuracy without increasing the output cost, while preserving the properties of the original model design and its optimal structure.